The power of ensemble methods was demonstrated in the preceding chapters. An ensemble with decision trees forms a homogeneous ensemble, and this was the main topic of Chapter 3, Bagging, to Chapter 6, Boosting Refinements. In Chapter 1, Introduction to Ensemble Techniques, and Chapter 7, The General Ensemble Technique, we had a peek at stacked ensembles. A central assumption in an ensemble is that the models are independent of one another. However, this assumption is seldom true, and we know that the same data partition is used over and over again. This does not mean that ensembling is bad; we have every reason to use the ensembles while previewing the concerns in an ensemble application. Consequently, we need to see how close the base models are to each other and overall in their predictions. If the predictions are close to each other, then we might need those base models in the ensemble. Here, we will build logistic regression, Naïve Bayes, SVM, and a decision...
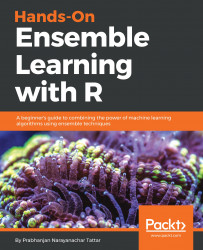
Hands-On Ensemble Learning with R
By :
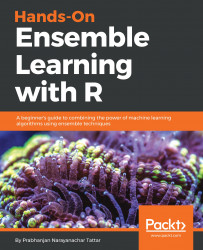
Hands-On Ensemble Learning with R
By:
Overview of this book
Ensemble techniques are used for combining two or more similar or dissimilar machine learning algorithms to create a stronger model. Such a model delivers superior prediction power and can give your datasets a boost in accuracy.
Hands-On Ensemble Learning with R begins with the important statistical resampling methods. You will then walk through the central trilogy of ensemble techniques – bagging, random forest, and boosting – then you'll learn how they can be used to provide greater accuracy on large datasets using popular R packages. You will learn how to combine model predictions using different machine learning algorithms to build ensemble models. In addition to this, you will explore how to improve the performance of your ensemble models.
By the end of this book, you will have learned how machine learning algorithms can be combined to reduce common problems and build simple efficient ensemble models with the help of real-world examples.
Table of Contents (17 chapters)
Hands-On Ensemble Learning with R
Contributors
Preface
Introduction to Ensemble Techniques
Bootstrapping
Bagging
Random Forests
The Bare Bones Boosting Algorithms
Boosting Refinements
The General Ensemble Technique
Ensemble Diagnostics
Ensembling Regression Models
Ensembling Survival Models
Ensembling Time Series Models
What's Next?
Bibliography
Index
Customer Reviews