In CNN networks, the way connectivity is defined among layers is significantly different compared to MLP or DBN. The convolutional (conv) layer is the main type of layer in a CNN, where each neuron is connected to a certain region of the input image, which is called a receptive field.
To be more specific, in a CNN architecture, a few conv layers are connected in a cascade style: each layer is followed by a rectified linear unit (ReLU) layer, then a pooling layer, then a few more conv layers (+ReLU), then another pooling layer, and so on. The output from each conv layer is a set of objects called feature maps, which are generated by a single kernel filter. Then, the feature maps are fed to the next layer as a new input. In the fully connected layer, each neuron produces an output followed by an activation layer (that is, the Softmax layer):
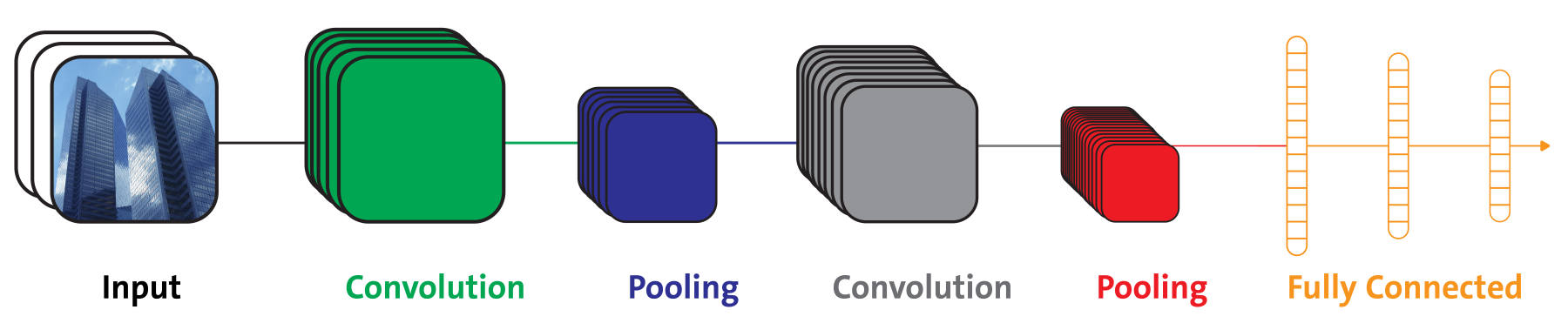