AI architectures on Azure
To understand the addition of AI components in a larger solution, we will take a look at some common architectures. Similar to the way data is ingested, machine learning predictions occur either in real time or in batches.
Let’s explore a sample architecture of each approach.
Scoring data in batches
The following figure is an example of a data architecture on Azure with batch scoring.
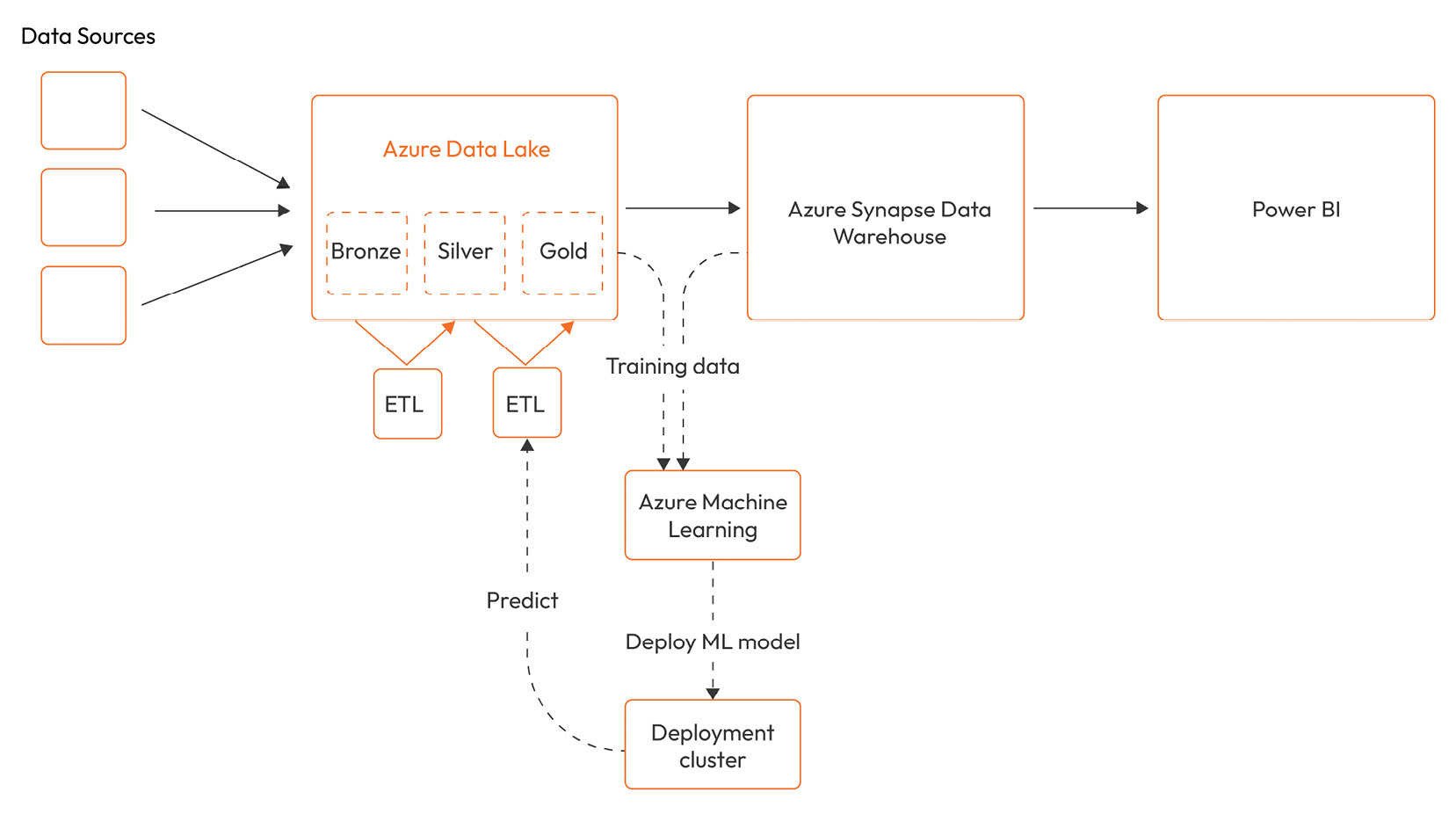
Figure 9.10 – An example data architecture involving batch scoring in the ETL process
Using data from the data lake and data warehouse, a custom model can be developed in the Azure Machine Learning workspace. The workspace does not copy data, so there are no additional storage costs. It will either mount data from the data lake or load tabular data straight into memory during training jobs in machine learning pipelines. When performing batch scoring, we do not want to deploy the model as an endpoint but, rather, have a deployed...