What is geospatial data science?
Geospatial data science lies at the intersection of data science and GIS as depicted in Figure 1.4:
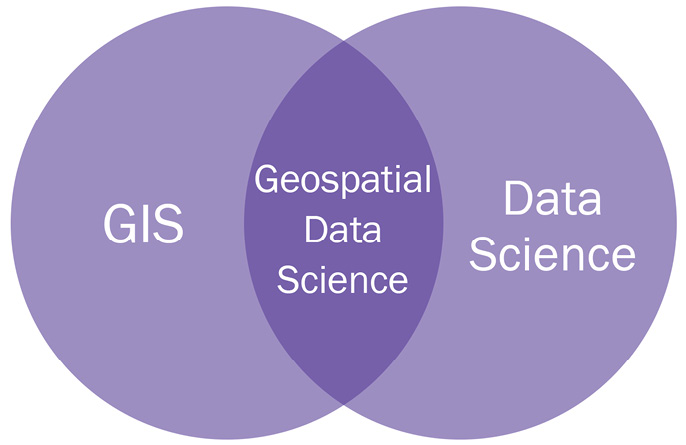
Figure 1.4 – Geospatial data science Venn diagram
Geospatial data science is a subclass of general data science that concentrates on geospatial data, its unique properties, and specialized techniques and computation methods necessary for deriving insights from this data. Instead of treating spatial data as another feature in a tabular dataset, spatial data science goes deeper into understanding why things are happening in a particular place and how they are related, or unrelated, to the things going on around it. Spatial data science focuses on identifying spatial relationships based on location, distance, and intersections between objects. We’ll talk more about spatial relationships in Part 2, Exploratory Spatial Data Analysis, of this book, as we conduct exploratory spatial data analysis.
While this book will focus primarily on geospatial data science, that is, data science focused on data pertaining to the Earth, it is worth noting that the concepts can be expanded and translated to general spatial data science. Spatial data science focuses on where the datum is located and how it is related across space and therefore can be applied to problems at a microscopic scale, such as the location of atoms in your body, or problems much larger, such as the distance between asteroids in the main belt between Mars and Jupiter.
By looking at data while taking into account its spatial relationships, you will often find substantial improvements to existing or developing models. As mentioned at the start of this chapter, the domain of spatial data science is rapidly growing and new avenues for exploration are uncovered every day.