6.2 Introducing approximate Bayesian inference via dropout
Dropout is traditionally used to prevent overfitting an NN. First introduced in 2012, it is now used in many common NN architectures and is one of the easiest and most widely used regularization methods. The idea of dropout is to randomly turn off (or drop) certain units of a neural network during training. Because of this, the model cannot solely rely on a particular small subset of neurons to solve the task it was given. Instead, the model is forced to find different ways to solve its task. This improves the robustness of the model and makes it less likely to overfit.
If we simplify a network to y = Wx, where y is the output of our network, x the input, and W our model weights, we can think of dropout as:
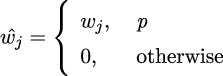
where wj is the new weights after applying dropout, wj is our weights before applying dropout, and p is our probability of not applying dropout.
The original dropout paper recommends randomly dropping 50% of the units in...