Moving beyond MLPs – RNNs
RNNs are a type of NN that is able to take into account the order of items in an input. In the example of the MLP that was discussed previously, the vector representing the entire input (that is, the complete document) was fed to the NN at once, so the network had no way of taking into account the order of words in the document. However, this is clearly an oversimplification in the case of text data since the order of words can be very important to the meaning. RNNs are able to take into account the order of words by using earlier outputs as inputs to later layers. This can be especially helpful in certain NLP problems where the order of words is very important, such as named entity recognition (NER), part-of-speech (POS) tagging, or slot labeling.
A diagram of a unit of an RNN is shown in Figure 10.5:
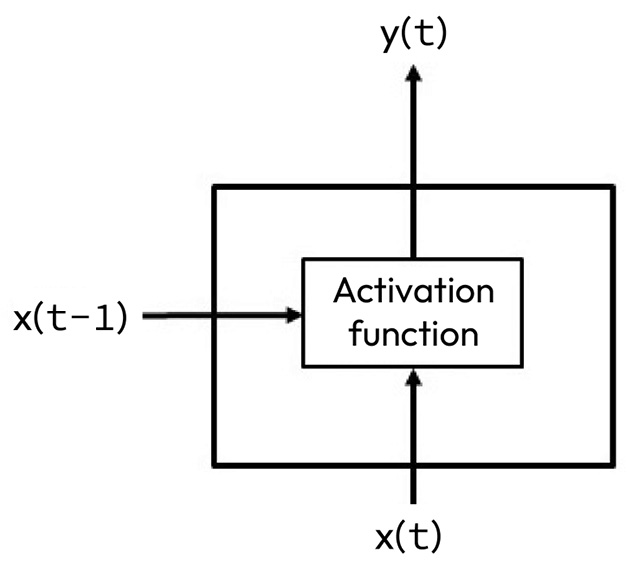
Figure 10.5 – A unit of an RNN
The unit is shown at time t. The input at time t, x(t), is passed to the activation...