Choosing among preprocessing techniques
Table 5.2 is a summary of the preprocessing techniques described in this chapter, along with their advantages and disadvantages. It is important for every project to consider which techniques will lead to improved results:
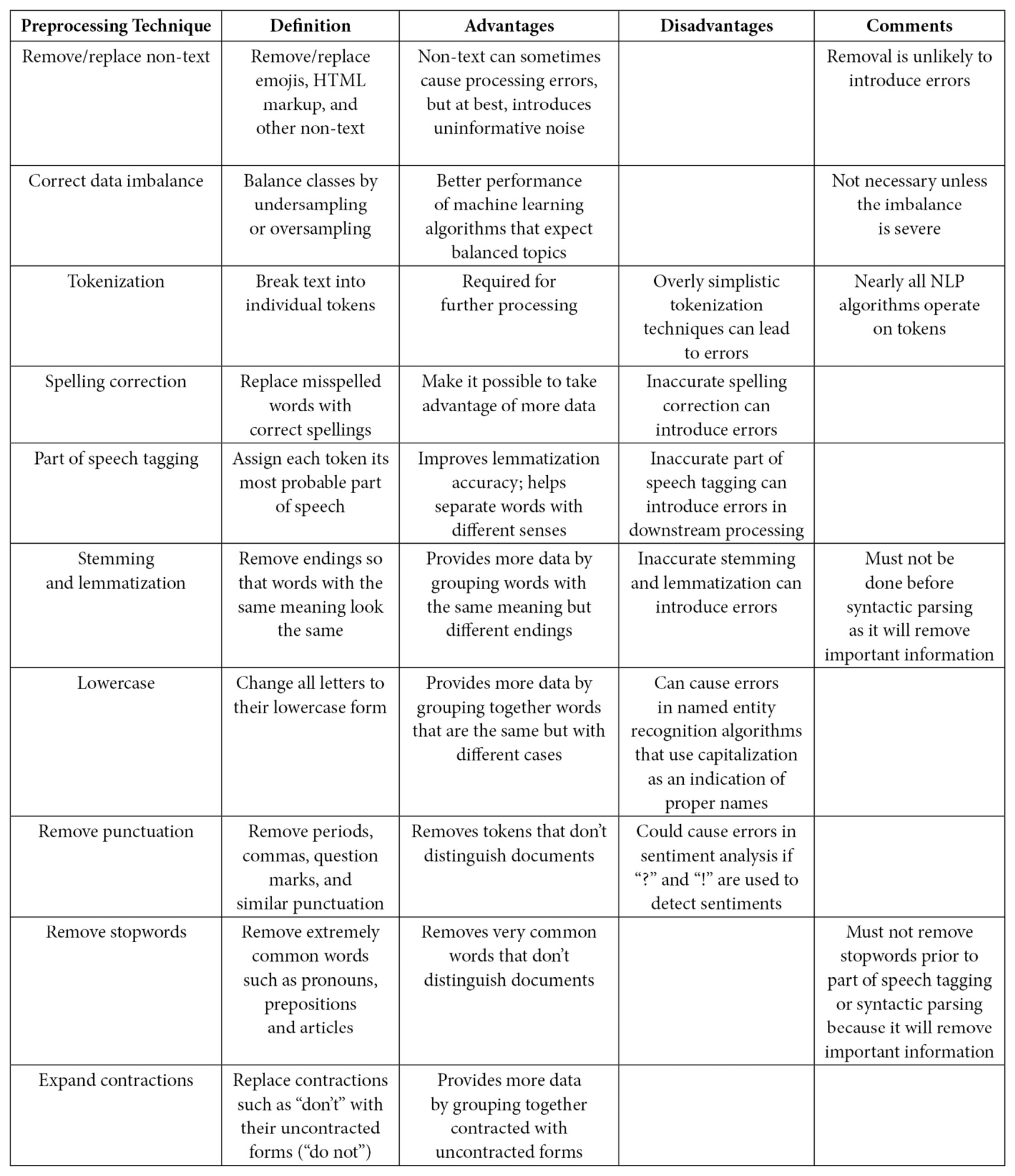
Table 5.2 – Advantages and disadvantages of preprocessing techniques
Many techniques, such as spelling correction, have the potential to introduce errors because the technology is not perfect. This is particularly true for less well-studied languages, for which the relevant algorithms can be less mature than those of better-studied languages.
It is worth starting with an initial test with only the most necessary techniques (such as tokenization) and introducing additional techniques only if the results of the initial test are not good enough. Sometimes, the errors introduced by preprocessing can cause the overall results to get worse. It is important to keep evaluating results during...