Advanced types of convolutions
So far, we’ve discussed the “classic” convolutional operation. In this section, we’ll introduce several new variations and their properties.
1D, 2D, and 3D convolutions
In this chapter, we’ve used 2D convolutions because computer vision with two-dimensional images is the most common CNN application. But we can also have 1D and 3D convolutions, where the units are arranged in one-dimensional or three-dimensional space, respectively. In all cases, the filter has the same number of dimensions as the input, and the weights are shared across the input. For example, we would use 1D convolution with time series data because the values are arranged across a single time axis. In the following diagram, on the left, we can see an example of 1D convolution:
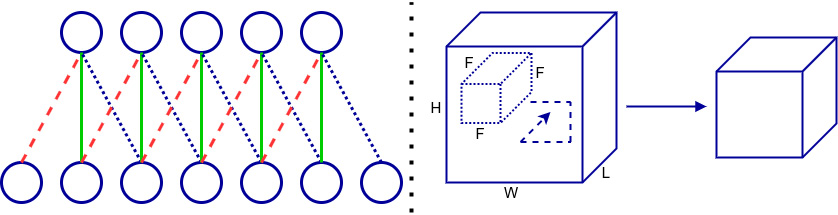
Figure 4.12 –1D convolution (left); 3D convolution (right)
The weights with the same dashed lines share the same value. The output...