Implementing text classification
In this section, we’ll use LSTM to implement a sentiment analysis example over the Large Movie Review Dataset (IMDb, http://ai.stanford.edu/~amaas/data/sentiment/), which consists of 25,000 training and 25,000 testing reviews of popular movies. Each review has a binary label that indicates whether it is positive or negative. This type of problem is an example of a many-to-one relationship, which we defined in the Recurrent neural networks (RNNs) section.
The sentiment analysis model is displayed in the following diagram:
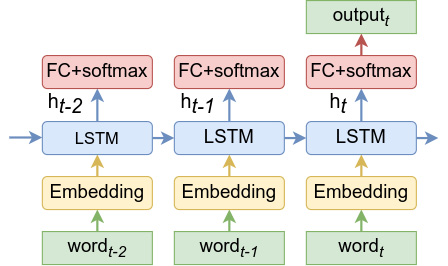
Figure 6.15 – Sentiment analysis with word embeddings and LSTM
Let’s describe the model components (these are valid for any text classification algorithm):
- Each word of the sequence is replaced with its embedding vector. These embeddings can be produced with word2vec.
- The word embedding is fed as input to the LSTM cell.
- The cell output,
, serves as input to an FC...