Starting with Spark 2.0, the performance of PySpark using DataFrames was on apar with that of Scala or Java. However, there was one exception: using User Defined Functions (UDFs); if a user defined a pure Python method and registered it as a UDF, under the hood, PySpark would have to constantly switch runtimes (Python to JVM and back). This was the main reason for an enormous performance hit compared with Scala, which does not need to convert the JVM object to a Python object.
Things have changed significantly in Spark 2.3. First, Spark started using the new Apache project. Arrow creates a single memory space used by all environments, thus removing the need for constant copying and converting between objects.
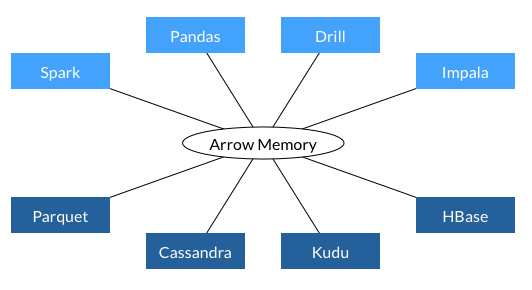
Source: https://arrow.apache.org/img/shared.png
Note
For an overview of Apache Arrow, go to https://arrow.apache.org.
Second, Arrow stores columnar objects in memory giving a big performance boost. Thus, in order to further leverage that, portions of the PySpark...