A draft genome assembly of a previously unsequenced genome can be a rich source of biological knowledge, but when genomics resources such as gene annotations aren't available, it can be tricky to proceed. Here, we'll look at a first stage pipeline for finding potential genes and genomic loci of interest absolutely de novo and without information beyond the sequence. We'll use a very simple set of rules to find open reading frames—sequences that begin with a start codon and end with a stop codon. The tools for doing this are encapsulated within a single function in the Bioconductor package, systemPipeR. We'll end up with yet another GRanges object that we can integrate into processes downstream that allow us to cross-reference other data, such as RNAseq, as we saw in the Finding unannotated transcribed...
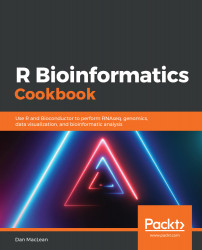
R Bioinformatics Cookbook
By :
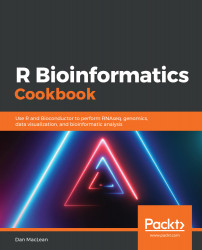
R Bioinformatics Cookbook
By:
Overview of this book
Handling biological data effectively requires an in-depth knowledge of machine learning techniques and computational skills, along with an understanding of how to use tools such as edgeR and DESeq. With the R Bioinformatics Cookbook, you’ll explore all this and more, tackling common and not-so-common challenges in the bioinformatics domain using real-world examples.
This book will use a recipe-based approach to show you how to perform practical research and analysis in computational biology with R. You will learn how to effectively analyze your data with the latest tools in Bioconductor, ggplot, and tidyverse. The book will guide you through the essential tools in Bioconductor to help you understand and carry out protocols in RNAseq, phylogenetics, genomics, and sequence analysis. As you progress, you will get up to speed with how machine learning techniques can be used in the bioinformatics domain. You will gradually develop key computational skills such as creating reusable workflows in R Markdown and packages for code reuse.
By the end of this book, you’ll have gained a solid understanding of the most important and widely used techniques in bioinformatic analysis and the tools you need to work with real biological data.
Table of Contents (13 chapters)
Preface
Performing Quantitative RNAseq
Finding Genetic Variants with HTS Data
Searching Genes and Proteins for Domains and Motifs
Phylogenetic Analysis and Visualization
Metagenomics
Proteomics from Spectrum to Annotation
Producing Publication and Web-Ready Visualizations
Working with Databases and Remote Data Sources
Useful Statistical and Machine Learning Methods
Programming with Tidyverse and Bioconductor
Building Objects and Packages for Code Reuse
Other Books You May Enjoy
Customer Reviews