A decision tree is simple yet powerful model for supervised learning problems. Like the name suggests, we can think of it as a tree in which information flows along different branches--starting at the trunk and going all the way to the individual leaves. If you are wondering if you have ever seen a decision tree before, let me remind you about the spam filter figure we encountered in Chapter 1, A Taste of Machine Learning:
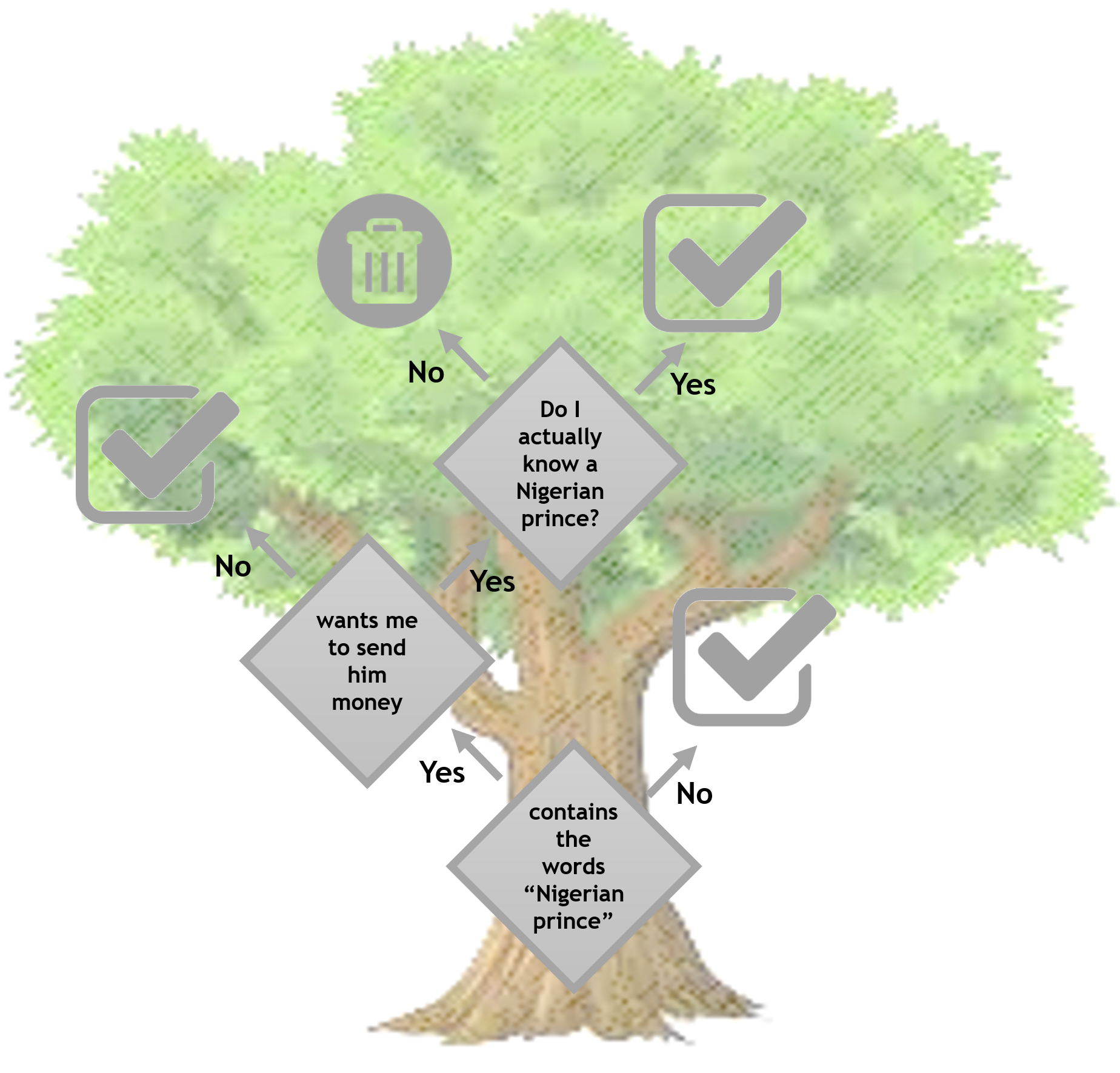
This is basically a decision tree!
A decision tree is made of a hierarchy of questions or tests about the data (also known as decision nodes) and their possible consequences. In the preceding example, we might count the number of words in each email using the CountVectorizer object from the previous chapter. Then it's easy to ask the first question (whether the email contains the words...