Apache Hadoop is the leading open source big data platform that can store and analyze massive amounts of structured and unstructured data efficiently and can be hosted on low cost commodity hardware. There are other technologies that complement Hadoop under the big data umbrella such as MongoDB, a NoSQL database; Cassandra, a document database; and VoltDB, an in-memory database. This section describes Apache Hadoop core concepts and its ecosystem.
Doug Cutting created Hadoop; he named it after his kid's stuffed yellow elephant and it has no real meaning. In 2004, the initial version of Hadoop was launched as Nutch Distributed Filesystem (NDFS). In February 2006, Apache Hadoop project was officially started as a standalone development for MapReduce and HDFS. By 2008, Yahoo adopted Hadoop as the engine of its Web search with a cluster size of around 10,000. In the same year, 2008, Hadoop graduated at top-level Apache project confirming its success. In 2012, Hadoop 2.x was launched with YARN, enabling Hadoop to take on various types of workloads.
Today, Hadoop is known by just about every IT architect and business executive as the open source big data platform and is used across all industries and sizes of organizations.
In this section, we will explore what Hadoop actually comprises. At the basic-level, Hadoop consists of the following four layers:
Hadoop Common: A set of common libraries and utilities used by Hadoop modules.
Hadoop Distributed File System (HDFS): A scalable and fault tolerant distributed filesystem to data in any form. HDFS can be installed on commodity hardware and replicates the data three times (which is configurable) to make the filesystem robust and tolerate partial hardware failures.
Yet Another Resource Negotiator (YARN): From Hadoop 2.0, YARN is the cluster management layer to handle various workloads on the cluster.
MapReduce: MapReduce is a framework that allows parallel processing of data in Hadoop. It breaks a job into smaller tasks and distributes the load to servers that have the relevant data. The framework effectively executes tasks on nodes where data is present thereby reducing the network and disk I/O required to move data.
The following figure shows you the high-level Hadoop 2.0 core components:
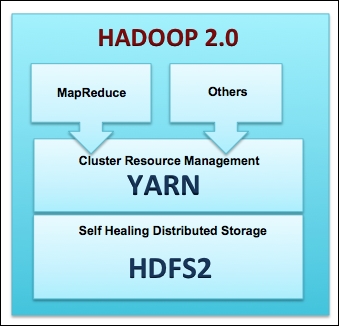
The preceding figure shows you the components that form the basic Hadoop framework. In past few years, a vast array of new components have emerged in the Hadoop ecosystem that take advantage of YARN making Hadoop faster, better, and suitable for various types of workloads. The following figure shows you the Hadoop framework with these new components:
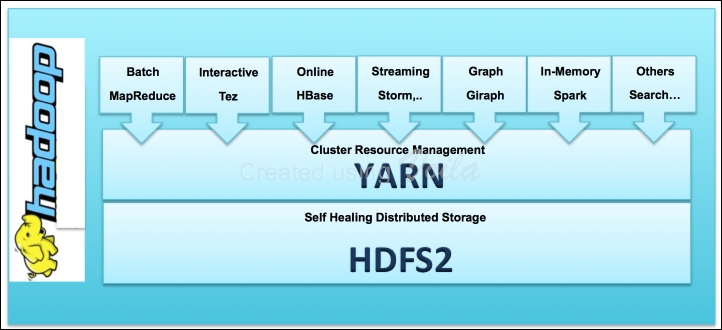
Each Hadoop cluster has the following two types of machines:
A network switch interconnects the master and worker nodes.
Note
It is recommended that you have separate servers for each of the master nodes; however, it is possible to deploy all the master nodes onto a single server for development or testing environments.
The following figure shows you the typical Hadoop cluster layout:
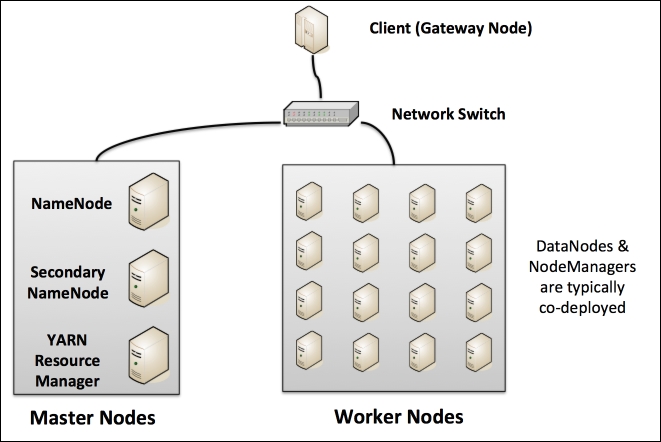
Let's review the key functions of the master and worker nodes:
NameNode: This is the master for the distributed filesystem and maintains metadata. This metadata has the listing of all the files and the location of each block of a file, which are stored across the various slaves. Without a NameNode, HDFS is not accessible. From Hadoop 2.0 onwards, NameNode HA (High Availability) can be configured with active and standby servers.
Secondary NameNode: This is an assistant to NameNode. It communicates only with NameNode to take snapshots of HDFS metadata at intervals that is configured at cluster level.
YARN ResourceManager: This server is a scheduler that allocates available resources in the cluster among the competing applications.
Worker nodes: The Hadoop cluster will have several worker nodes that handle two types of functions: HDFS DataNode and YARN NodeManager. It is typical that each worker node handles both these functions for optimal data locality. This means that processing happens on the data that is local to the node and follows the principle "move code and not data".
This section will look into the distributed filesystem in detail. The following figure shows you a Hadoop cluster with four data nodes and NameNode in HA mode. The NameNode is the bookkeeper for HDFS and keeps track of the following details:
List of all files in HDFS
Blocks associated with each file
Location of each block including the replicated blocks
Starting with HDFS 2.0, NameNode is no longer a single point of failure that eliminates any business impact in case of hardware failures.
Note
Secondary NameNode is not required in NameNode HA configuration, as the Standby NameNode performs the tasks of the Secondary NameNode.
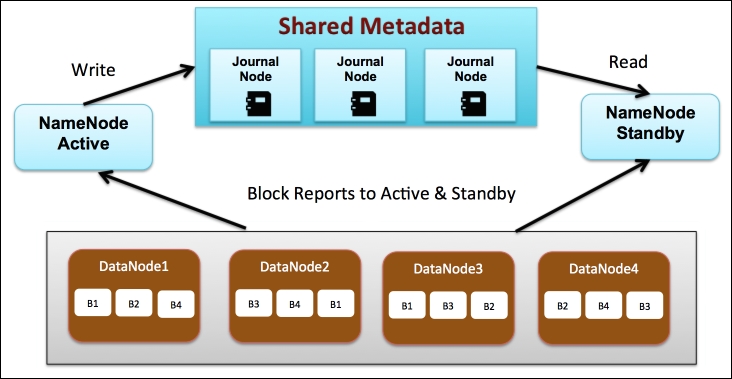
Next, let's review how data is written and read from HDFS.
When a file is ingested to Hadoop, it is first divided into several blocks where each block is typically 64 MB in size that can be configured by administrators. Next, each block is replicated three times onto different data nodes for business continuity so that even if one data node goes down, the replicas come to the rescue. The replication factor is configurable and can be increased or decreased as desired. The preceding figure shows you an example of a file called MyBigfile.txt
that is split into four blocks B1, B2, B3, and B4. Each block is replicated three times across different data nodes.
The active NameNode is responsible for all client operations and writes information about the new file and blocks the shared metadata and the standby NameNode reads from this shared metadata. The shared metadata requires a group of daemons called journal nodes.
When a request to read a file is made, the active NameNode refers to the shared metadata in order to identify the blocks associated with the file and the locations of those blocks. In our example, the large file, MyBigfile.txt
, the NameNode will return a location for each of the four blocks B1, B2, B3, and B4. If a particular data node is down, then the nearest and not so busy replica's block is loaded.
Let's look at the commonly used Hadoop commands used to access the distributed filesystem:
Command |
Syntax |
---|---|
Listing of files in a directory |
|
Create a new directory |
|
Copy a file from a local machine to Hadoop |
|
Copy a file from Hadoop to a local machine |
|
Tail last few lines of a large file in Hadoop |
|
View the complete contents of a file in Hadoop |
|
Remove a complete directory from Hadoop |
|
Check the Hadoop filesystem space utilization |
|
Note
For a complete list of Hadoop commands, refer to the link http://hadoop.apache.org/docs/current/hadoop-project-dist/hadoop-common/FileSystemShell.html.
Now that we are able to save the large file, the next obvious need would be to process this file and get something useful out of it such as a summary report. Hadoop YARN, which stands for Yet Another Resource Manager, is designed for distributed data processing and is the architectural center of Hadoop. This area in Hadoop has gone through a major rearchitecturing in Version 2.0 of Hadoop and YARN has enabled Hadoop to be a true multiuse data platform that can handle batch processing, real-time streaming, interactive SQL, and is extensible for other custom engines. YARN is flexible, efficient, provides resource sharing, and is fault-tolerant.
YARN consists of a central ResourceManager that arbitrates all available cluster resources and per-node NodeManagers that take directions from the ResourceManager and are responsible for managing resources available on a single node. NodeManagers have containers that perform the real computation.
ResourceManager has the following main components:
Scheduler: This is responsible for allocating resources to various running applications, subject to constraints of capacities and queues that are configured
Applications Manager: This is responsible for accepting job submissions, negotiating the first container for executing the application, which is called "Application Master"
NodeManager is the worker bee and is responsible for managing containers, monitoring their resource usage (CPU, memory, disk, and network), and reporting the same to the ResourceManager. The two types of containers present are as follows:
Application Master: This is one per application and has the responsibility of negotiating with appropriate resource containers from the ResourceManager, tracking their status, and monitoring their progress.
Application Containers: This gets launched as per the application specifications. An example of an application is MapReduce, which is used for batch processing.
Let's understand how the various components in YARN actually interact with a walkthrough of an application lifecycle. The following figure shows you a Hadoop cluster with one master ResourceManager and four worker NodeManagers:
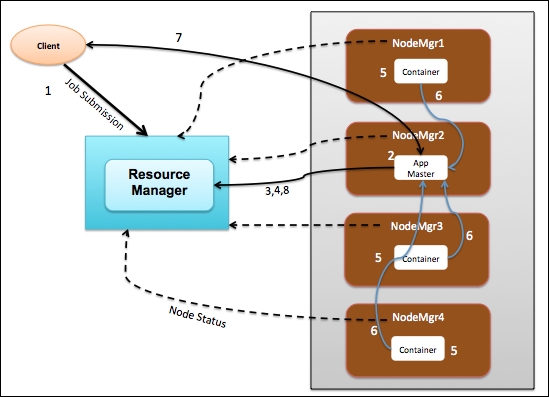
Let's walkthrough the sequence of events in a life of an application such as MapReduce job:
The client program submits an application request to the ResourceManager and provides the necessary specifications to launch the application.
The ResourceManager takes over the responsibility to identify a container to be started as an Application Master and then launches the Application Master, which in our case is NodeManager 2 (NodeMgr2).
The Application Master on boot-up registers with the ResourceManager. This allows the client program to get visibility on which Node is handling the Application Master for further communication.
The Application Master negotiates with the ResourceManager for containers to perform the actual tasks. In the preceding figure, the application master requested three resource containers.
On successful container allocations, the Application Master launches the container by providing the specifications to the NodeManager.
The application code executing within the container provides status and progress information to the Application Master.
During the application execution, the client who submits the program communicates directly with the Application Master to get status, progress, and updates.
After the application is complete, the Application Master deregisters with the ResourceManager and shuts down, allowing all the containers associated with that application to be repurposed.
Prior to Hadoop 2.0, MapReduce was the standard approach to process data on Hadoop. With the introduction of YARN, which has a flexible architecture, various other types of workload are now supported and are now great alternatives to MapReduce with better performance and management. Here is a list of commonly used workloads on top of YARN:
The combination of HDFS, which is a distributed data store, and YARN, which is a flexible data operating system, make Hadoop a true multiuse data platform enabling modern data architecture.