We began this chapter with the intuition that a good model is one that effectively explains the data and is also simple. Using this intuition, we discussed the problem of overfitting and underfitting that pervades statistical and machine learning practices. We then formalized our intuitions by introducing the concept of deviance and information criteria. We started with the rather unsophisticated AIC, and its more Bayesian cousin known as DIC. Then we learned about an improved version of both, WAIC. We also discussed briefly the empirical cross-validation method and a way to approximate its results using the LOO method. We briefly discussed priors and hierarchical models in the light of the new ideas exposed in this chapter. Finally, we ended with a discussion of Bayes factors, how to compute them, and how to solve some usual sampling problems associated with them. We finished with an example to clarify the different aims of Bayes factors and information criteria.
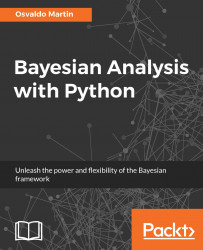
Bayesian Analysis with Python
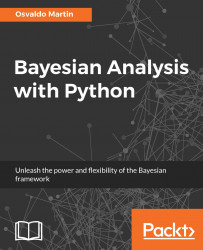
Bayesian Analysis with Python
Overview of this book
The purpose of this book is to teach the main concepts of Bayesian data analysis. We will learn how to effectively use PyMC3, a Python library for probabilistic programming, to perform Bayesian parameter estimation, to check models and validate them. This book begins presenting the key concepts of the Bayesian framework and the main advantages of this approach from a practical point of view. Moving on, we will explore the power and flexibility of generalized linear models and how to adapt them to a wide array of problems, including regression and classification. We will also look into mixture models and clustering data, and we will finish with advanced topics like non-parametrics models and Gaussian processes. With the help of Python and PyMC3 you will learn to implement, check and expand Bayesian models to solve data analysis problems.
Table of Contents (15 chapters)
Bayesian Analysis with Python
Credits
About the Author
About the Reviewer
www.PacktPub.com
Preface
Thinking Probabilistically - A Bayesian Inference Primer
Programming Probabilistically – A PyMC3 Primer
Juggling with Multi-Parametric and Hierarchical Models
Understanding and Predicting Data with Linear Regression Models
Classifying Outcomes with Logistic Regression
Model Comparison
Mixture Models
Gaussian Processes
Index
Customer Reviews