In the kernelized regression example, try changing the number of knots and the bandwidth (one at a time). What is the effect of those changes? Try also using a single knot; what do you observe?
Experiment with fitting other functions using kernelized regression. For example y = np.sin(x) + x**0.7 or y = x. Using these functions changes the number of data points and parameters like in Exercise 1
In the example where we sample from the GP prior increase the number or realizations, by replacing:
plt.plot(test_points, stats.multivariate_normal.rvs(cov=cov, size=6).T)
with
plt.plot(test_points, stats.multivariate_normal.rvs(cov=cov, size=1000).T, alpha=0.05, color='b')
How does the GP prior look? Do you see that f(x) is distributed as a Gaussian centered at 0 and standard deviation 1?
For the GP posterior using the Gaussian kernel, try defining
test_points
outside the interval [0, 10]. What happened outside thedata interval
? What does this tell us about extrapolating results (especially for...
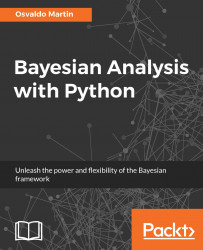
Bayesian Analysis with Python
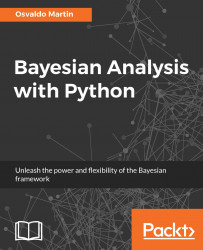
Bayesian Analysis with Python
Overview of this book
The purpose of this book is to teach the main concepts of Bayesian data analysis. We will learn how to effectively use PyMC3, a Python library for probabilistic programming, to perform Bayesian parameter estimation, to check models and validate them. This book begins presenting the key concepts of the Bayesian framework and the main advantages of this approach from a practical point of view. Moving on, we will explore the power and flexibility of generalized linear models and how to adapt them to a wide array of problems, including regression and classification. We will also look into mixture models and clustering data, and we will finish with advanced topics like non-parametrics models and Gaussian processes. With the help of Python and PyMC3 you will learn to implement, check and expand Bayesian models to solve data analysis problems.
Table of Contents (15 chapters)
Bayesian Analysis with Python
Credits
About the Author
About the Reviewer
www.PacktPub.com
Preface
Thinking Probabilistically - A Bayesian Inference Primer
Programming Probabilistically – A PyMC3 Primer
Juggling with Multi-Parametric and Hierarchical Models
Understanding and Predicting Data with Linear Regression Models
Classifying Outcomes with Logistic Regression
Model Comparison
Mixture Models
Gaussian Processes
Index
Customer Reviews