So, it's time to start with the simplest yet still very useful abstraction for our data–a linear regression function.
In linear regression, we try to find a linear equation that minimizes the distance between the data points and the modeled line. The model function takes the following form:
yi = ßxi +α+εi
Here, α is the intercept and ß is the slope of the modeled line. The variable x is normally called the independent variable, and y the dependent one, but it can also be called the regressor and the response variables.
The εi variable is a very interesting element, and it's the error or distance from the sample i to the regressed line.
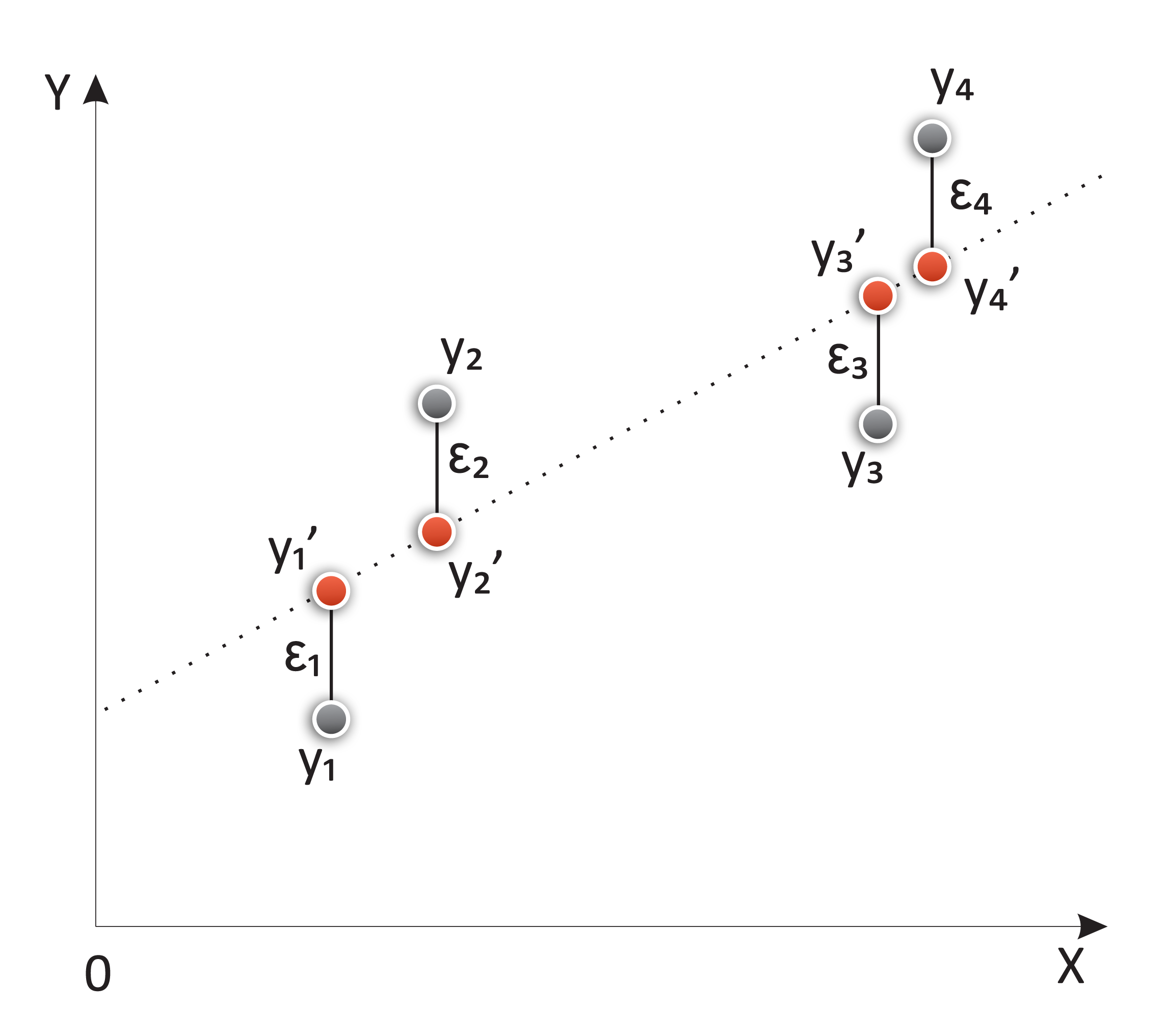
Depiction of the components of a regression line, including the original elements, the estimated ones (in red), and the error (ε)
The set of all those distances, calculated...