Let's start this chapter with the possible applications of generative models. The applications are enormous. We will see a few of these applications to understand the motivation and possibilities.
Artistic style transfer is the process of transferring the style of art to any image. For example, an image can be created with the artistic style of an image and content of another image. An example of one image combined with several different styles is shown here illustrated by Gatys et al. (https://www.cv-foundation.org/openaccess/content_cvpr_2016/papers/Gatys_Image_Style_Transfer_CVPR_2016_paper.pdf). The image A is the photo on which the style is applied, and the results are shown in other images:
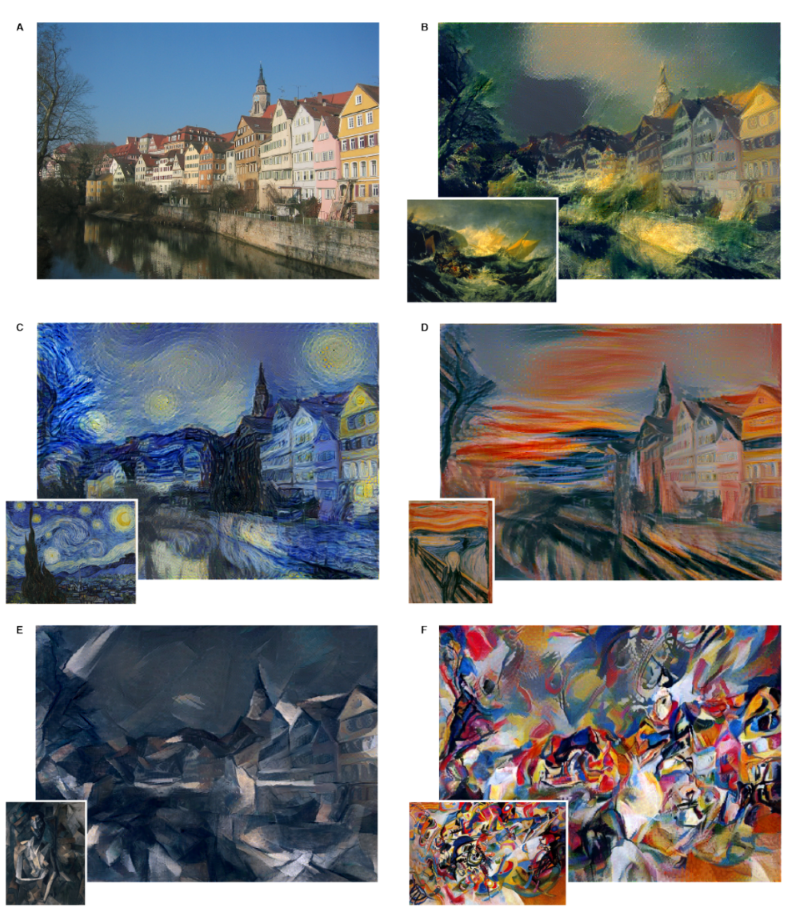
Reproduced from Gatys et al.
This application has caught the public's attention, and there are several mobile apps in the market providing this facility.