In the case that the input data is sparse or if we want fast convergence while training complex neural networks, we get the best results using adaptive learning rate methods. We also don't need to tune the learning rate. For most cases, Adam is usually a good choice.
Let's take an example of linear regression, where we try to find the best fit for a straight line through a number of data points by minimizing the squares of the distance from the line to each data point. This is why we call it least squares regression. Essentially, we are formulating the problem as an optimization problem, where we are trying to minimize a loss function.
Let's set up input data and look at the scatter plot:
# input data xData = np.arange(100, step=.1) yData = xData + 20 * np.sin(xData/10)
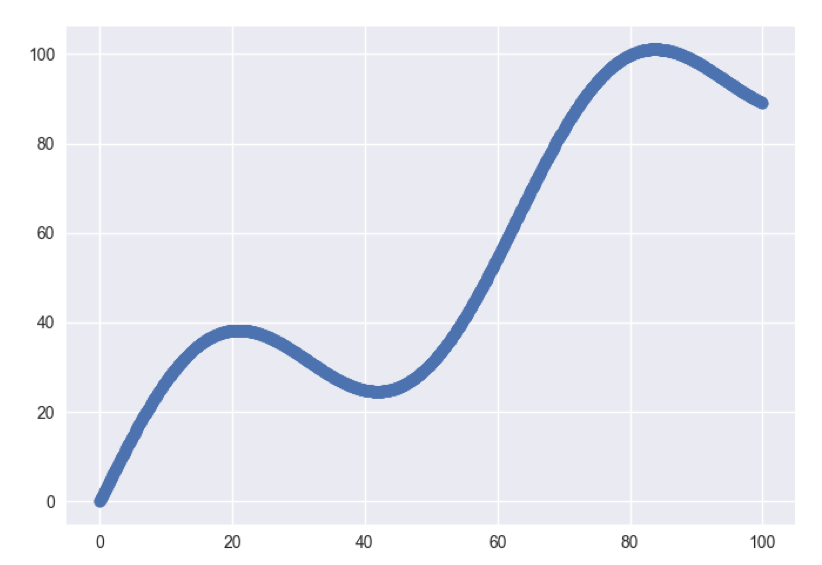
Define the data size and batch size:
# define the data size and batch size
nSamples = 1000
batchSize = 100
We will need to resize the data to meet the TensorFlow input format...