Convolutional layers are often intertwined with pooling layers, which down sample the output of the previous convolutional layer in order to decrease the amount of parameters we need to compute. A particular form of these layers, max pooling layers, has become the most widely used variant. In general terms, max pooling layers tell us if a feature was present in the region, the previous convolutional layer was looking at; it looks for the most significant value in a particular region (the maximum value), and utilizes that value as a representation of the region, as shown as follows:
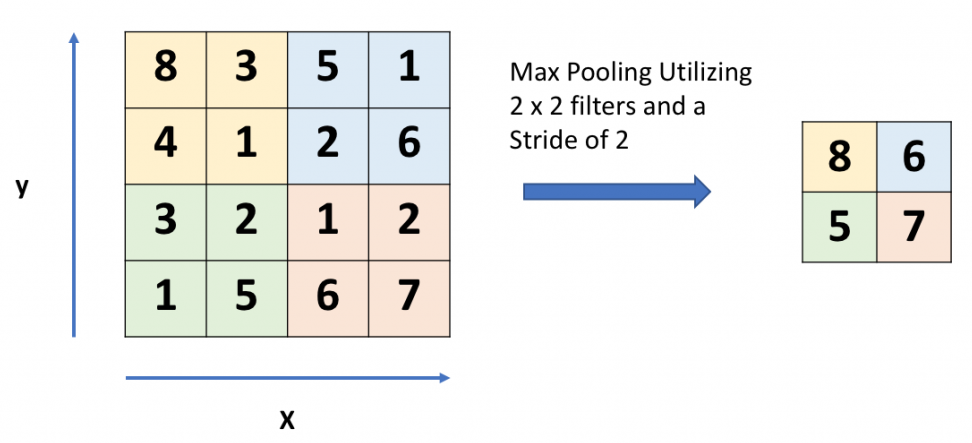
Max pooling layers help subsequent convolutional layers focus on larger sections of the data, providing abstractions of the that help both reduce overfitting and the amount of hyperparameters that we have to learn, ultimately reducing our computational cost. This form of automatic feature...