In this chapter, we introduced recurrent neural networks and demonstrated how to use an RNN on the MNIST dataset. RNNs are particularly useful for working with time series data, since they are essentially feedforward networks that are unrolled over time. This makes them very suitable for tasks such as handwriting and speech recognition, as they operate on sequences of data. We also looked at a more powerful variant of the RNN, the LSTM. The LSTM uses four gates to decide what information to pass on to the next time step, enabling it to uncover long-term dependencies in data. Finally, in this chapter we built a simple language model, enabling us to generate text from sample input text. We used a model based on the GRU. The GRU is a slightly simplified version of the LSTM, containing three gates and combining the input and forget gates of the LSTM. This model used probability...
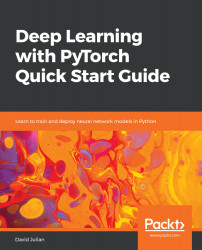
Deep Learning with PyTorch Quick Start Guide
By :
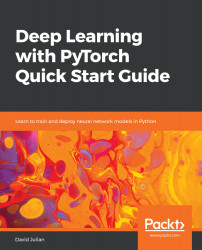
Deep Learning with PyTorch Quick Start Guide
By:
Overview of this book
PyTorch is extremely powerful and yet easy to learn. It provides advanced features, such as supporting multiprocessor, distributed, and parallel computation. This book is an excellent entry point for those wanting to explore deep learning with PyTorch to harness its power.
This book will introduce you to the PyTorch deep learning library and teach you how to train deep learning models without any hassle. We will set up the deep learning environment using PyTorch, and then train and deploy different types of deep learning models, such as CNN, RNN, and autoencoders.
You will learn how to optimize models by tuning hyperparameters and how to use PyTorch in multiprocessor and distributed environments. We will discuss long short-term memory network (LSTMs) and build a language model to predict text.
By the end of this book, you will be familiar with PyTorch's capabilities and be able to utilize the library to train your neural networks with relative ease.
Table of Contents (8 chapters)
Preface
Introduction to PyTorch
Deep Learning Fundamentals
Computational Graphs and Linear Models
Convolutional Networks
Other NN Architectures
Getting the Most out of PyTorch
Other Books You May Enjoy
Customer Reviews