Use cases in healthcare
As we delve into the ethical use of AI in the healthcare industry, we should know how the early detection and treatment of diseases relate to ethical AI. Here are a few examples:
- AI can support diagnosis using X-rays, CT scans, and MRI imaging techniques.
- Detecting cancers, tumors, and other malignant cells in the early stages of development.
- Experimenting with and determining whether a treatment is working.
- Monitoring patients to identify the reoccurrence or remission of a disease.
The following figure illustrates how AI-based deep learning algorithms can help identify the presence of an IDH1 gene mutation in a brain tumor after being trained on images that radiologists and doctors have labeled as suspected cancer (https://www.cancer.gov/news-events/cancer-currents-blog/2022/artificial-intelligence-cancer-imaging):
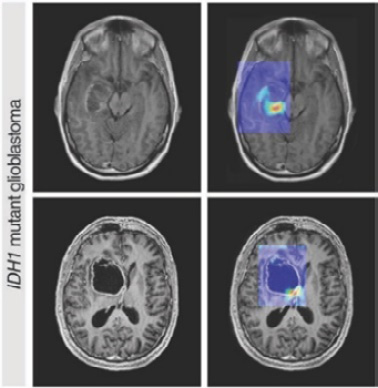
Figure 14.12 – MRI scans predicting the presence of an IDH1 gene mutation in brain...