Reviewing backpropagation explainability
Feature attribution methods generally encompass two steps to determine inference results through a forward pass, followed by a backpropagation to assign relevance scores for input features on a trained model.
Backpropagation is a gradient-based XAI method that evaluates feature attributions by generating partial derivatives of output in multiple forward and backward passes through neural networks. Unlike neural network training, backpropagation in the context of feature attribution does not require gradient computation for weight updates on model parameters. Instead of beginning with the input layer, backpropagation feature attribution starts by assigning importance scores at the final output layer, then calculating local activation gradients inversely across each intermediate layer until it reaches the input layer, as shown in Figure 7.2:
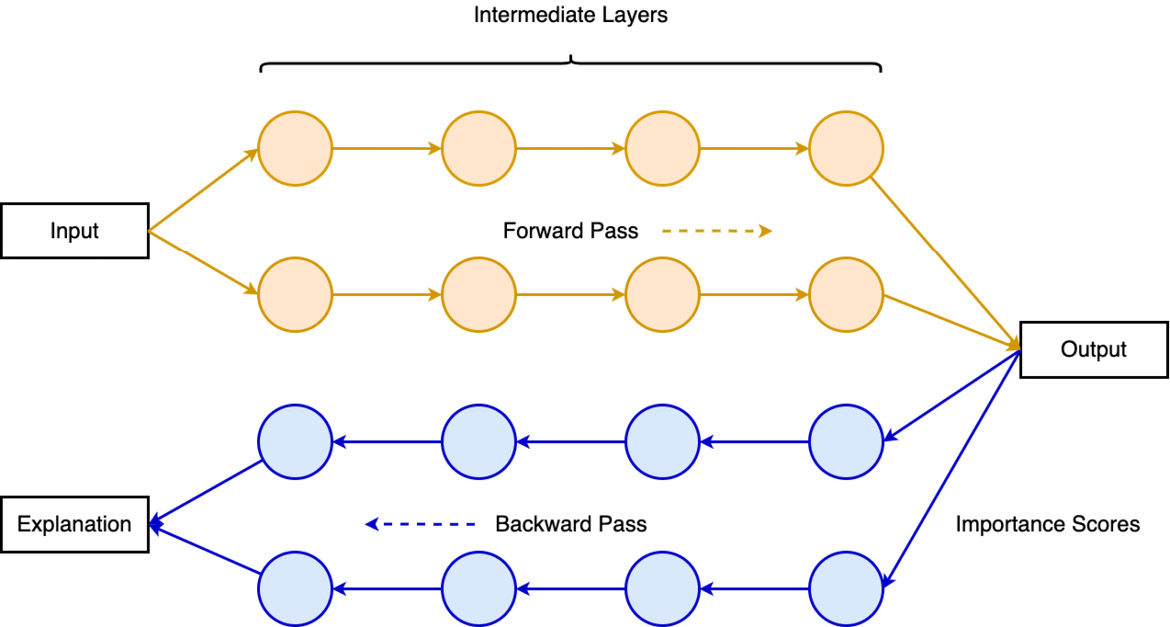
Figure 7.2 – Backpropagation XAI