Natural Language Processing Anomaly Explainability
The evolution of Natural Language Processing (NLP) began in the 1950s with machine translation converting Russian into English. The advent of computing power and big data motivated NLP innovation, leading to a new subfield of linguistics, computer science, and artificial intelligence. Figure 3.1 shows the intersections of NLP with other disciplines:
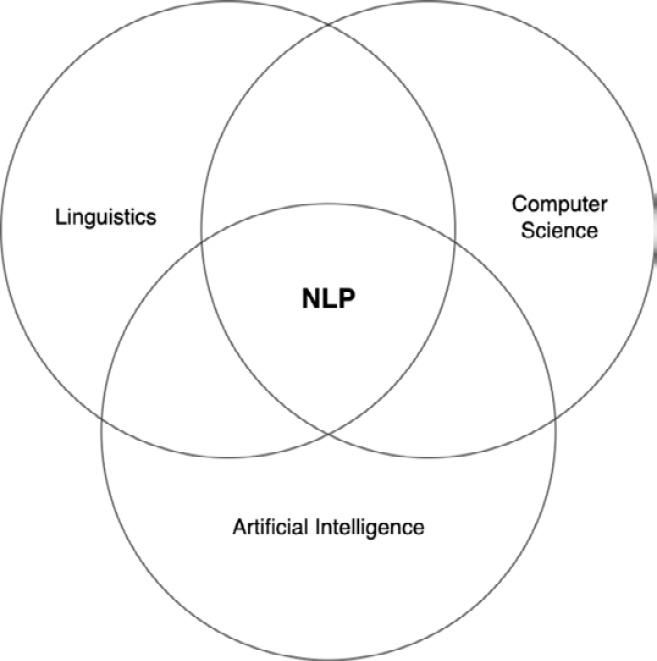
Figure 3.1 – NLP Venn diagram
There is abundant unstructured textual data around us for NLP tasks, such as text classification, topic modeling, and intent detection. NLP enables computers to understand and analyze massive amounts of written and verbal human language at scale. The goal is to contextualize language nuances and extract useful information to derive valuable insights.
Deep learning has revolutionized many NLP applications, including text summarization, machine translation, and question-answering. However, despite high accuracy, industry...