Bringing it all together
Start with updating the frontend code with the HTTP address for face-detection-app as in the refreshFaceCounter
and takepicture
functions. Keep in mind that your URL will be different.
Save and load the HTML file into your browser. The browser will throw a warning that the application is trying to capture video feed; allow the application to access the video feed. You will get a screen like the one shown in Figure 7.23.
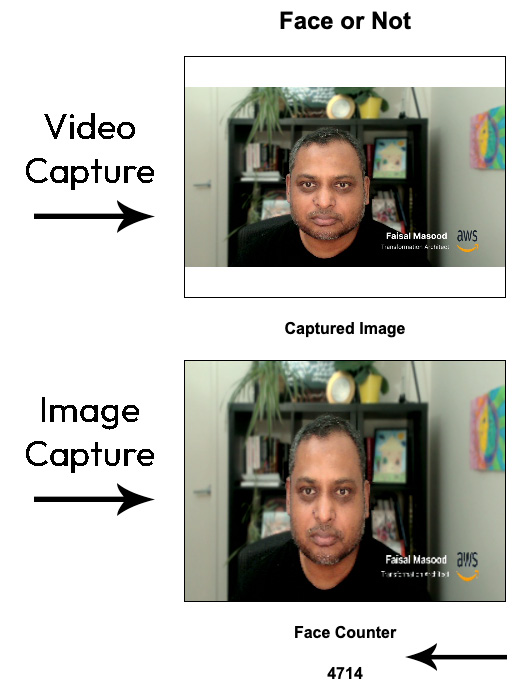
Figure 7.23 – Application UI capturing video and inferencing
The web page captures the video stream from your laptop camera and displays it in the top area of the page. The middle area shows the image capture every 250 milliseconds as configured on the web page, and the bottom counter displays the number of images captured.
You will notice that the counter is continuously incremented while the person sits in front of the camera. This means that every 250 milliseconds, an image has been captured and...