In this section, we will look at SVMs, what they are, and how they classify data. We will discuss important hyperparameters, including how kernel methods are used. Finally, we will see their use by training them on the Titanic dataset.
With SVMs, we seek to find a hyperplane that best separates instances of two classes. These classes are assumed to be linearly separable. All data on one side of the hyperplane is predicted to belong to one class. All others belong to the other class. By best line, we mean that the plane separates classes while at the same time maximizing the distance between the line and the nearest data point, as shown in the following diagram:
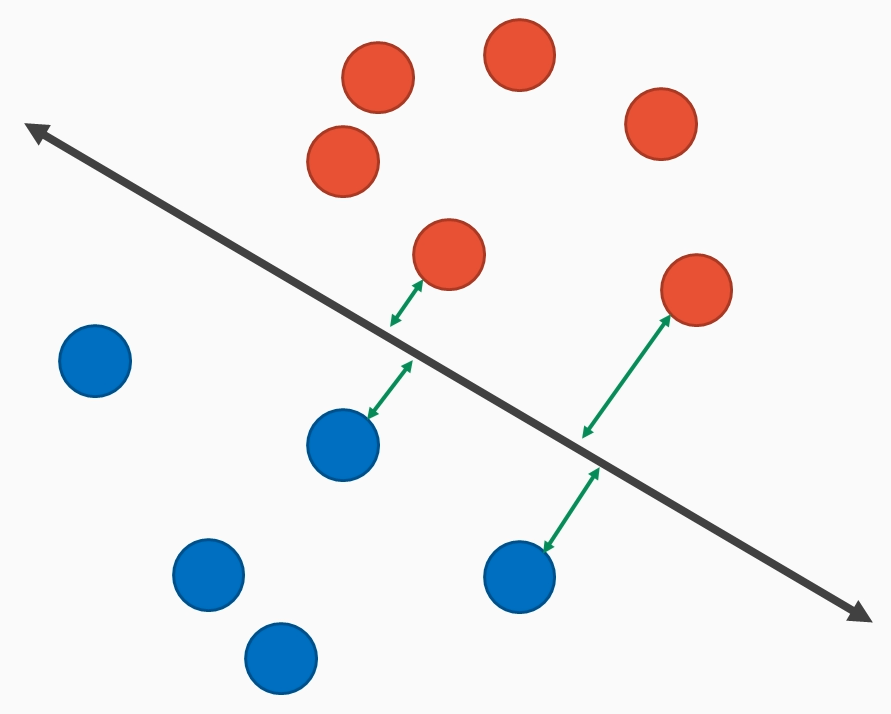
The hope is that by doing this, the SVM will generalize well to data that hasn't been seen. There are two hyperparameters that are of interest to SVMs:
- One is a tolerance parameter of C that...