This section focuses on the class of classifiers and regression algorithms known as neural networks. In this section, we will cover the theory behind neural networks. We will also look at one specific type of neural network—that is, the MLP.
Neural networks are inspired by a model of how the brain works. In this model, a neuron is connected with other neurons in the brain; if the signals surpass a threshold, then the neuron is induced to activate. This is better illustrated in the following diagram:
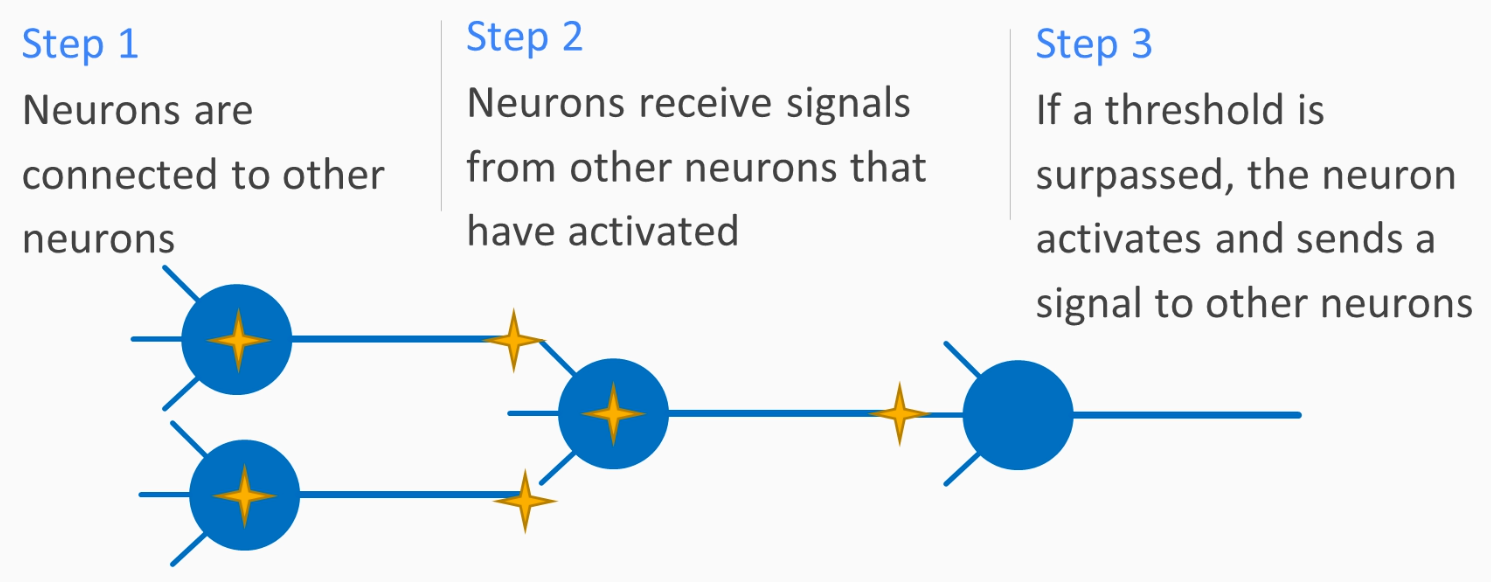
The neuron that is activated is then passed to other neurons, spreading messages. Neural networks in machine learning emulate this process; neural networks are characterized by how information is transmitted through the network.