15.5 Extras
Here are some ideas for you to add to this project.
15.5.1 Measures of shape
The measurements of shape often involve two computations for skewness and kurtosis. These functions are not part of Python’s built-in statistics
library.
It’s important to note that there are a very large number of distinct, well-understood distributions of data. The normal distribution is one of many different ways data can be distributed.
See https://www.itl.nist.gov/div898/handbook/eda/section3/eda366.htm.
One measure of skewness is the following:
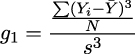
Where Ȳ is the mean, and s is the standard deviation.
A symmetric distribution will have a skewness, g1, near zero. Larger numbers indicate a ”long tail” opposite a large concentration of data around the mean.
One measure of kurtosis is the following:
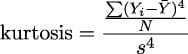
The kurtosis for the standard normal distribution is 3. A value larger than 3 suggests more data is in the tails; it’s ”flatter” or ”...