DL basics
DL was introduced in 2012. The basic idea is to mimic the human brain and construct artificial neural networks (ANNs) to train models. A typical multi-layer ANN has three types of layers: an input layer, one or more hidden layers, and an output layer. Figure 6.15 shows an ANN that has one input layer, two hidden layers, and an output layer. In the ANN, a circular node represents a perceptron, and a line represents the connection from the output of one perceptron to the input of another.
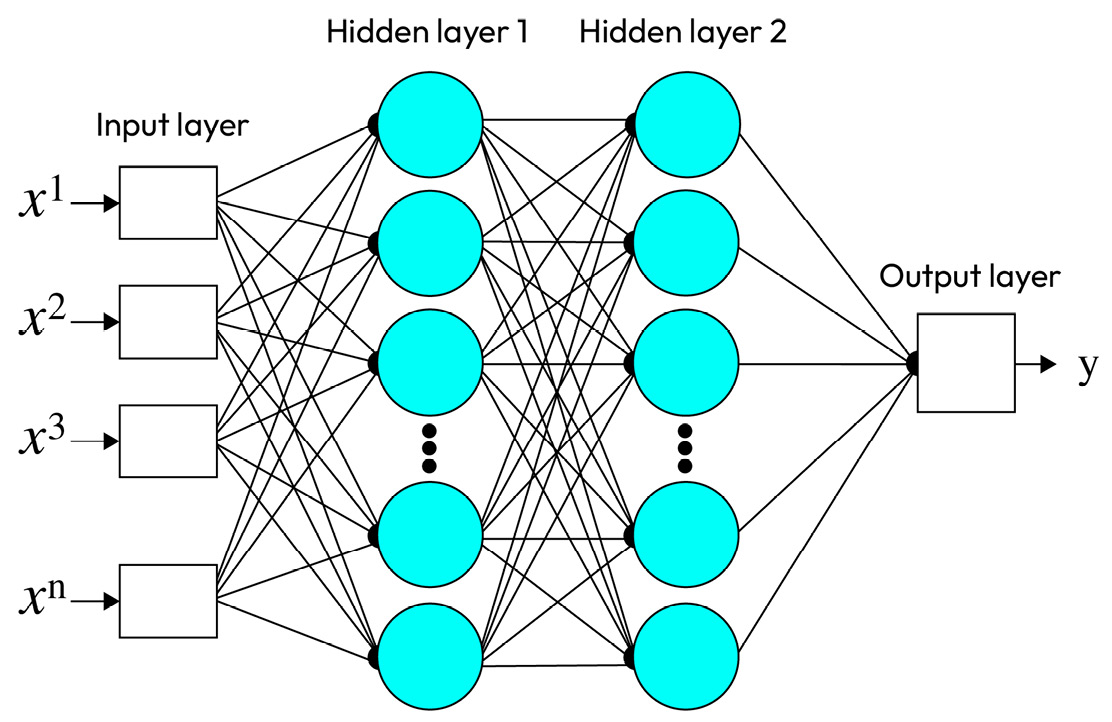
Figure 6.15 – A multi-layer ANN
The objective of DL model training is the same as ML: minimize the loss function, which is defined as the gap between the model’s predicted value and the actual value. Different from traditional ML algorithms, DL uses the activation function to add nonlinearity to the model training process.
In a typical DL model, we define the following to construct a neural network:
- The layers of the model (input layer...