You already saw unstructured data in the previous chapters, the data was an array of LabeledPoint, which is a tuple (label: Double, features: Vector). The label is just a number of type Double. Vector is a sealed trait with two subclasses: SparseVector and DenseVector. The class diagram is as follows:
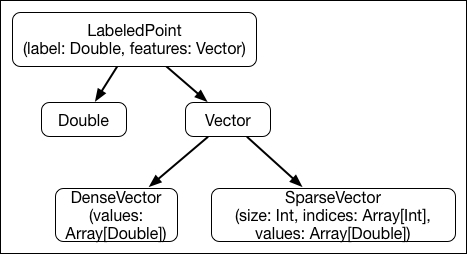
Figure 1: The LabeledPoint class structure is a tuple of label and features, where features is a trait with two inherited subclasses {Dense,Sparse}Vector. DenseVector is an array of double, while SparseVector stores only size and non-default elements by index and value.
Each observation is a tuple of label and features, and features can be sparse. Definitely, if there are no missing values, the whole row can be represented as vector. A dense vector representation requires (8 x size + 8) bytes. If most of the elements are missing—or equal to some default value—we can store only the non-default elements. In this case, we would require (12 x non_missing_size + 20) bytes, with small...