ML – a predictive tool
Working through a predictive model involves optimization at multiple steps on top of optimally fitting the learning algorithm to the data. It involves transforming raw data into a form most appropriate for consumption in learning algorithms. An ML model has hyperparameters that can be configured to tailor it to a specific dataset. It is a standard practice to test a suite of hyper-parameters for a chosen ML algorithm, which is called hyper-parameter tuning or optimization. A grid search or random search algorithm is used for such tuning. Figure 2.6 shows the two search algorithm types. Grid search is more suitable for a quick search of hyperparameters and is known to perform well in general. You can also use Bayesian optimization for hyper-parameter tuning in some problems. We will learn about these optimization techniques in detail in the last part of the book.
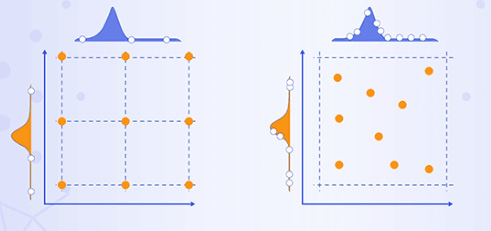
Figure 2.6: Grid search (L) versus random search (R)
An ML practitioner...