Optimization use case
Graphs can be used to model relations and processes in physical, biological, and information systems. They have a wide range of applications, such as ranking hyperlinks in search engines, the study of biomolecules, computer network security, GPS in maps to find the shortest route, and social network analysis. There are knowledge graphs for information mining as well. In the following subsection, we pick a dataset and formulate the problem in a way that is solved using graph theory.
Optimization problem
There can be multiple paths between origin and destination airports. An airline seeks the shortest possible path between airports, wherein the shortest path can be defined in terms of either distance or airtime. If the city airports are represented as nodes and the flight routes between them as edges, we convert the problem into a graph (Figure 6.8a). The dataset can be found in the GitHub repository: https://github.com/ranja-sarkar/graphs.
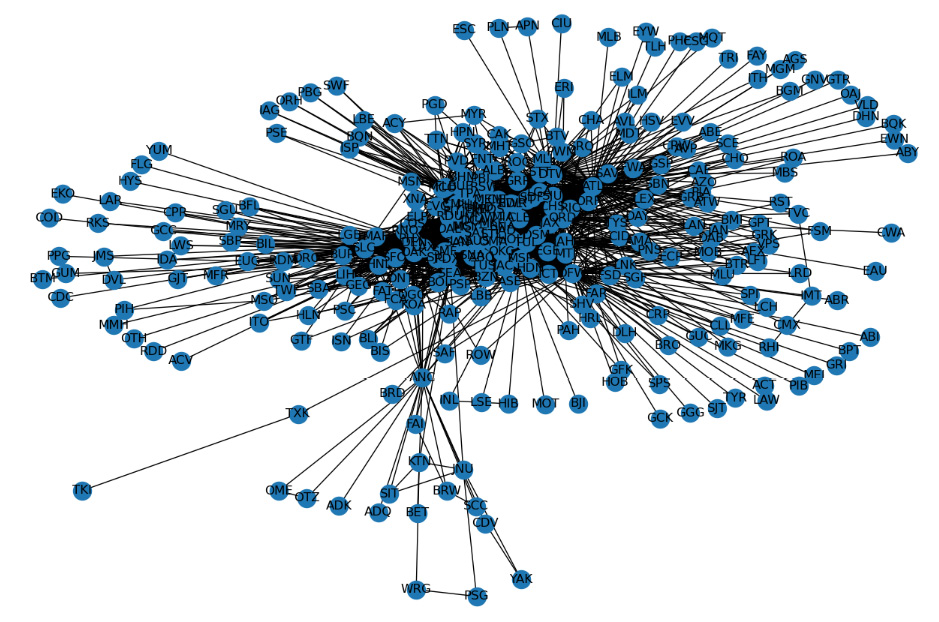