Computation of measurements
We will start with a flow diagram of the Kalman filter algorithm, shown in Figure 7.3a. The Kalman filter requires an initial guess to start with. This input can be a very rough estimate. So, step 0 is the initial guess and step 1 is the measurement of the state variable.
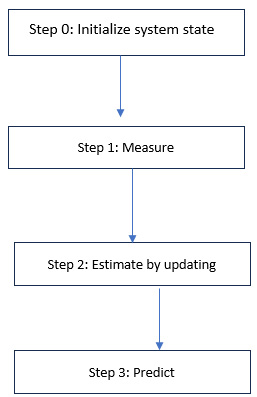
Figure 7.3a: Flow diagram of the Kalman filter
When the input is a measured value, the output is the current state estimated using the state update equation in step 2, which is calculated from the predicted value of the current state and the residual scaled (updated) by a factor called the Kalman gain. The Kalman gain takes the input measurement uncertainty into account, the residual being the difference between the measured and predicted values. This update and estimate make the second step in the algorithm.
The output from step 2 is fed to predict the next state of the system. The state for the next iteration is predicted using the dynamic model. The prediction...