Auto-encoders are a form of dimensionality reduction technique. When they are used in this manner, they mathematically and conceptually have similarities to other dimensionality reduction techniques such as PCA. Auto-encoders consist of two parts: an encoder which creates a representation of the data, and a decoder which tries to reproduce or predict the inputs. Thus, the hidden layers and neurons are not maps between an input and some other outcome, but are self (auto)-encoding. Given sufficient complexity, auto-encoders can simply learn the identity function, and the hidden neurons will exactly mirror the raw data, resulting in no meaningful benefit. Similarly, in PCA, using all the principal components also provides no benefit. Therefore, the best auto-encoder is not necessarily the most accurate one, but one that reveals some meaningful structure or architecture in the data or one that reduces noise, identifies outliers, or anomalous data, or some other useful...
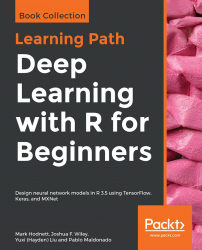
Deep Learning with R for Beginners
By :
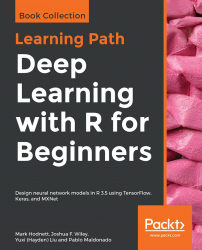
Deep Learning with R for Beginners
By:
Overview of this book
Deep learning has a range of practical applications in several domains, while R is the preferred language for designing and deploying deep learning models.
This Learning Path introduces you to the basics of deep learning and even teaches you to build a neural network model from scratch. As you make your way through the chapters, you’ll explore deep learning libraries and understand how to create deep learning models for a variety of challenges, right from anomaly detection to recommendation systems. The Learning Path will then help you cover advanced topics, such as generative adversarial networks (GANs), transfer learning, and large-scale deep learning in the cloud, in addition to model optimization, overfitting, and data augmentation. Through real-world projects, you’ll also get up to speed with training convolutional neural networks (CNNs), recurrent neural networks (RNNs), and long short-term memory networks (LSTMs) in R.
By the end of this Learning Path, you’ll be well-versed with deep learning and have the skills you need to implement a number of deep learning concepts in your research work or projects.
Table of Contents (23 chapters)
Title Page
Copyright and Credits
About Packt
Contributors
Preface
Getting Started with Deep Learning
Training a Prediction Model
Deep Learning Fundamentals
Training Deep Prediction Models
Image Classification Using Convolutional Neural Networks
Tuning and Optimizing Models
Natural Language Processing Using Deep Learning
Deep Learning Models Using TensorFlow in R
Anomaly Detection and Recommendation Systems
Running Deep Learning Models in the Cloud
The Next Level in Deep Learning
Handwritten Digit Recognition using Convolutional Neural Networks
Traffic Signs Recognition for Intelligent Vehicles
Fraud Detection with Autoencoders
Text Generation using Recurrent Neural Networks
Sentiment Analysis with Word Embedding
Other Books You May Enjoy
Index
Customer Reviews