In the previous section, we explained how to increase the receptive field of the neurons by using a stride larger than 1. But we can also do this with the help of pooling layers. A pooling layer splits the input slice into a grid, where each grid cell represents a receptive field of a number of neurons (just as a convolutional layer does). Then, a pooling operation is applied over each cell of the grid. Different types of pooling layers exist. Pooling layers don't change the volume depth, because the pooling operation is performed independently on each slice.
Max pooling: is the most popular way of pooling. The max pooling operation takes the neuron with the highest activation value in each local receptive field (grid cell), and propagates only that value forward. In the following figure, we can see an example of max pooling with a receptive field of 2x2:
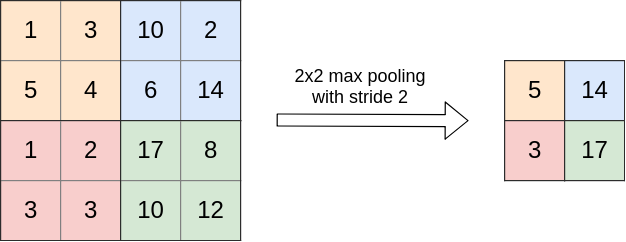