In this chapter, we discussed the concepts behind unsupervised and semi-supervised machine learning, and their Bayesian treatment. We learned two important Bayesian unsupervised models: the Bayesian mixture model and LDA. We discussed in detail the bgmm package for the Bayesian mixture model, and the topicmodels and lda packages for topic modeling. Since the subject of unsupervised learning is vast, we could only cover a few Bayesian methods in this chapter, just to give a flavor of the subject. We have not covered semi-supervised methods using both item labeling and feature labeling. Interested readers should refer to more specialized books in this subject. In the next chapter, we will learn another important class of models, namely neural networks.
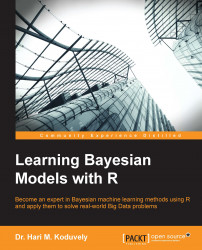
Learning Bayesian Models with R
By :
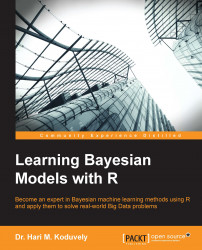
Learning Bayesian Models with R
By:
Overview of this book
Table of Contents (16 chapters)
Learning Bayesian Models with R
Credits
About the Author
About the Reviewers
www.PacktPub.com
Preface
Introducing the Probability Theory
The R Environment
Introducing Bayesian Inference
Machine Learning Using Bayesian Inference
Bayesian Regression Models
Bayesian Classification Models
Bayesian Models for Unsupervised Learning
Bayesian Neural Networks
Bayesian Modeling at Big Data Scale
Index
Customer Reviews