A typical neural network for a digit recognizer may have 784 input pixels connected to 1,000 neurons in the hidden layer, which in turn connects to 10 output targets — one for each digit. Each layer is fully connected to the layer above. A graphical representation of this network is shown as follows, where x are the inputs, h are the hidden neurons, and y are the output class variables:
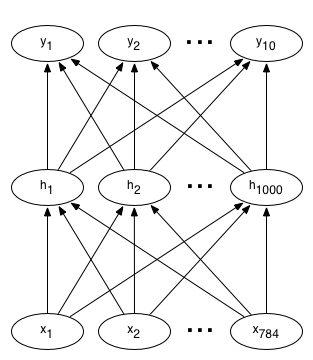
In this notebook, we will build a neural network that will recognize handwritten numbers from 0-9.
The type of neural network that we are building is used in a number of real-world applications, such as recognizing phone numbers and sorting postal mail by address. To build this network, we will use the MNIST dataset.
We will begin as shown in the following code by importing all the required modules, after which the data will be loaded, and then finally building the network:
# Import Numpy, keras and MNIST data import numpy as np import matplotlib.pyplot as plt from keras.datasets import mnist from keras.models import Sequential from keras.layers.core import Dense, Dropout, Activation from keras.utils import np_utils