In this example, we will take a pre-trained VGGNet and use transfer learning to train a CNN classifier that predicts dog breeds, given a dog image. Keras contains many pre-trained models, along with the code that loads and visualizes them. Another is a flower dataset that can be downloaded here. The Dog breed dataset has 133 dog breed categories and 8,351 dog images. Download the Dog breed dataset here and copy it to your folder. VGGNet has 16 convolutional with pooling layers from beginning to end and three fully connected layers followed by a softmax function. Its main objective was to show how the depth of the network gives the best performance. It came from Visual Geometric Group (VGG) at Oxford. Their best performing network is VGG16. The Dog breed dataset is relatively small and has a little overlap with the imageNet dataset. So, we can remove...
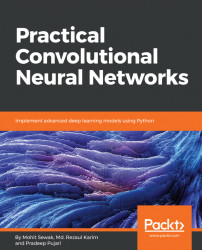
Practical Convolutional Neural Networks
By :
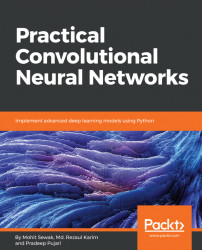
Practical Convolutional Neural Networks
By:
Overview of this book
Convolutional Neural Network (CNN) is revolutionizing several application domains such as visual recognition systems, self-driving cars, medical discoveries, innovative eCommerce and more.You will learn to create innovative solutions around image and video analytics to solve complex machine learning and computer vision related problems and implement real-life CNN models.
This book starts with an overview of deep neural networkswith the example of image classification and walks you through building your first CNN for human face detector. We will learn to use concepts like transfer learning with CNN, and Auto-Encoders to build very powerful models, even when not much of supervised training data of labeled images is available.
Later we build upon the learning achieved to build advanced vision related algorithms for object detection, instance segmentation, generative adversarial networks, image captioning, attention mechanisms for vision, and recurrent models for vision.
By the end of this book, you should be ready to implement advanced, effective and efficient CNN models at your professional project or personal initiatives by working on complex image and video datasets.
Table of Contents (11 chapters)
Preface
Deep Neural Networks – Overview
Introduction to Convolutional Neural Networks
Build Your First CNN and Performance Optimization
Popular CNN Model Architectures
Transfer Learning
Autoencoders for CNN
Object Detection and Instance Segmentation with CNN
GAN: Generating New Images with CNN
Attention Mechanism for CNN and Visual Models
Other Books You May Enjoy
Customer Reviews