In 2010, a challenge from ImageNet (known as ILSVRC 2010) came out with a CNN architecture, LeNet 5, built by Yann Lecun. This network takes a 32 x 32 image as input, which goes to the convolution layers (C1) and then to the subsampling layer (S2). Today, the subsampling layer is replaced by a pooling layer. Then, there is another sequence of convolution layers (C3) followed by a pooling (that is, subsampling) layer (S4). Finally, there are three fully connected layers, including the OUTPUT layer at the end. This network was used for zip code recognition in post offices. Since then, every year various CNN architectures were introduced with the help of this competition:
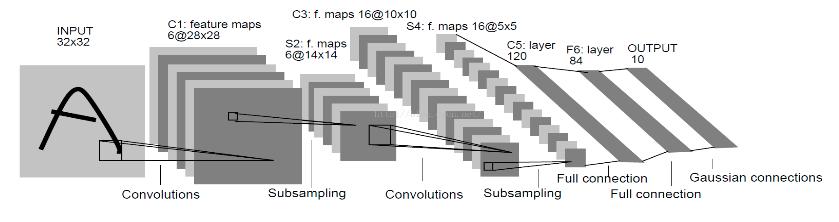
LeNet 5 – CNN architecture from Yann Lecun's article in 1998
Therefore, we can conclude the following points:
- The input to this network is a grayscale 32 x 32 image
- The architecture...