The CartPole system is a classic problem of reinforced learning. The system consists of a pole (which acts like an inverted pendulum) attached to a cart via a joint, as shown in the following diagram:
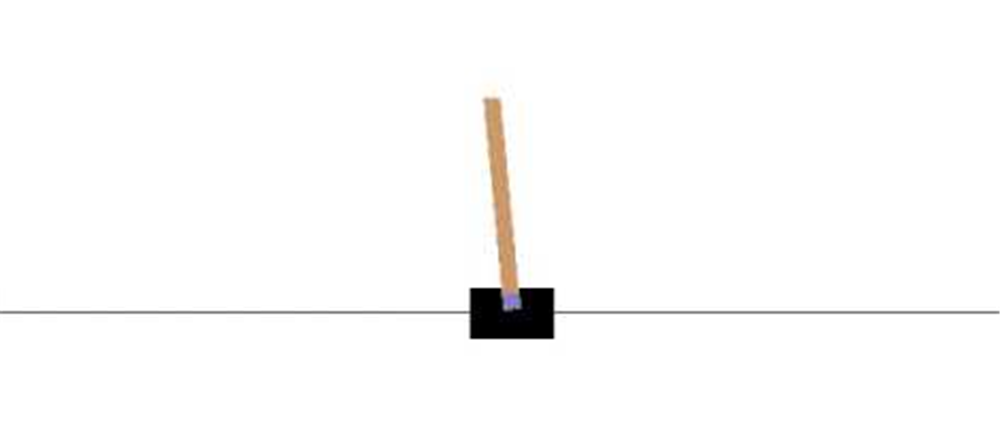
The system is controlled by applying a force of +1 or -1 to the cart. The force applied to the cart can be controlled, and the objective is to swing the pole upward and stabilize it. This must be done without the cart falling to the ground. At every step, the agent can choose to move the cart left or right, and it receives a reward of 1 for every time step that the pole is balanced. If the pole ever deviates by more than 15 degrees from upright, the procedure ends.
To run the CartPole example using the OpenAI Gym library, simply type the following code:
import gym
env = gym.make('CartPole-v0')
env.reset()
for i in range(1000):
env.render()
env.step...