To allow the model to have a more flexible separating hyperplane, all scikit-learn implementations are based on a simple variant that includes so-called slack variables ζi in the function to minimize (sometimes called C-Support Vector Machines):
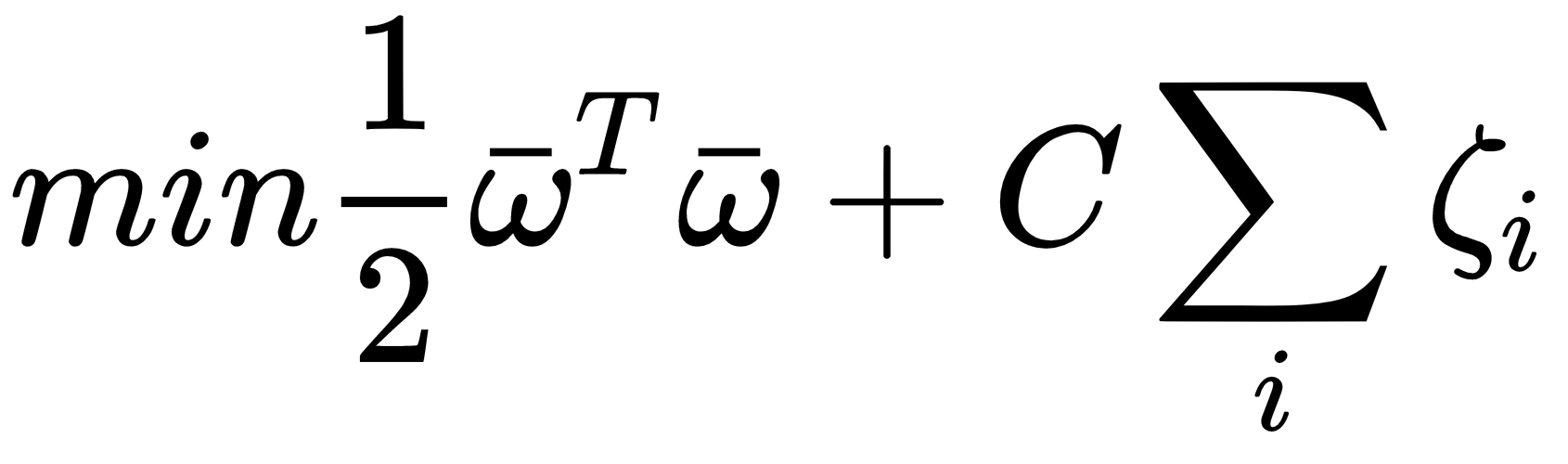
In this case, the constraints become the following:

The introduction of slack variables allows us to create a flexible margin so that some vectors belonging to a class can also be found in the opposite part of the hyperspace and can be included in the model training. The strength of this flexibility can be set using the C parameter. Small values (close to zero) bring about very hard margins, while values greater than or equal to 1 allow more and more flexibility (while also increasing the misclassification rate). Equivalently, when C → 0, the number of support vectors is minimized, while larger C values...