Behold, the LSTM architecture. This model, iconic in its use of complex information paths and gates, is capable of learning informative time dependent representations from the inputs it is shown. Each line in the following diagram represents the propagation of an entire vector from one node to another in the direction denoted by the arrows. When these lines split, the value they carry is copied to each pathway. Memory from previous time steps are shown to enter from the top-left of the unit, while activations from previous timesteps enter from the bottom-left corner.
The boxes represent the dot products of learned weight matrices and some inputs passed through an activation function. The circles represent point-wise operations, such as element-wise vector multiplication (*) or addition (+):
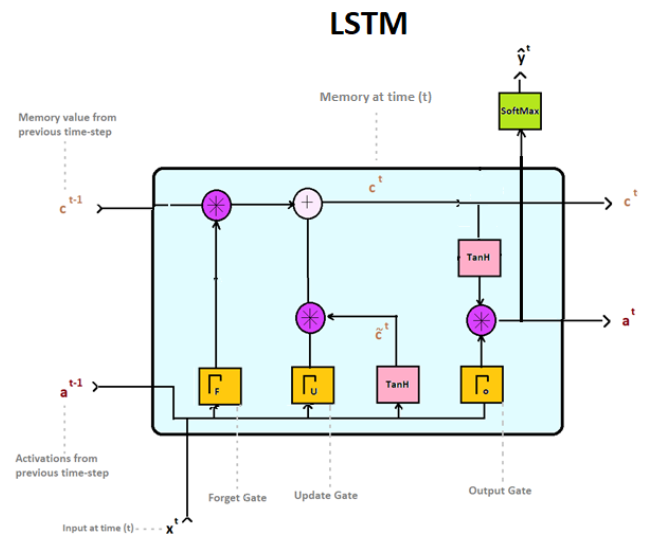
In the last chapter, we saw how RNNs may use a feedback connection through...