First things first, we will need a simulated environment. An environment is defined as the interaction space for a learning agent. For humans, an environment can be any play you go to in the course of a day. For an artificial agent, this will often be a simulated environment that we have engineered. Why is it simulated? Well, we could ask the agent to learn in real time, like ourselves, but it turns out that this is quite impractical. For one, we would have to design each agent a body and then precisely engineer its actions and the environments that they are to interact with. Moreover, an agent can train much faster in a simulation, without requiring it to be restricted to human time frames. By the time a machine completes a single task in reality, its simulated version could have completed the same task several times over, providing a better opportunity...
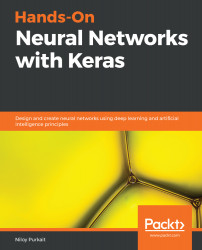
Hands-On Neural Networks with Keras
By :
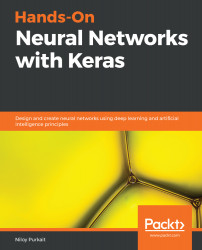
Hands-On Neural Networks with Keras
By:
Overview of this book
Neural networks are used to solve a wide range of problems in different areas of AI and deep learning.
Hands-On Neural Networks with Keras will start with teaching you about the core concepts of neural networks. You will delve into combining different neural network models and work with real-world use cases, including computer vision, natural language understanding, synthetic data generation, and many more. Moving on, you will become well versed with convolutional neural networks (CNNs), recurrent neural networks (RNNs), long short-term memory (LSTM) networks, autoencoders, and generative adversarial networks (GANs) using real-world training datasets. We will examine how to use CNNs for image recognition, how to use reinforcement learning agents, and many more. We will dive into the specific architectures of various networks and then implement each of them in a hands-on manner using industry-grade frameworks.
By the end of this book, you will be highly familiar with all prominent deep learning models and frameworks, and the options you have when applying deep learning to real-world scenarios and embedding artificial intelligence as the core fabric of your organization.
Table of Contents (16 chapters)
Preface
Overview of Neural Networks
A Deeper Dive into Neural Networks
Signal Processing - Data Analysis with Neural Networks
Section 2: Advanced Neural Network Architectures
Convolutional Neural Networks
Recurrent Neural Networks
Long Short-Term Memory Networks
Reinforcement Learning with Deep Q-Networks
Section 3: Hybrid Model Architecture
Autoencoders
Generative Networks
Section 4: Road Ahead
Contemplating Present and Future Developments
Other Books You May Enjoy
Customer Reviews