Defining explanation methods and approaches
In this section, let's try to understand some key concepts required for understanding and applying various explainability techniques and approaches.
Dimensions of explainability
Adding to the concepts presented at MICCAI'18 from Tutorial on Interpretable Machine Learning by Samek and Binder, when we talk about the problem of demystifying black-box algorithms, there are four different dimensions through which we can address this problem, as can be seen in the following diagram:
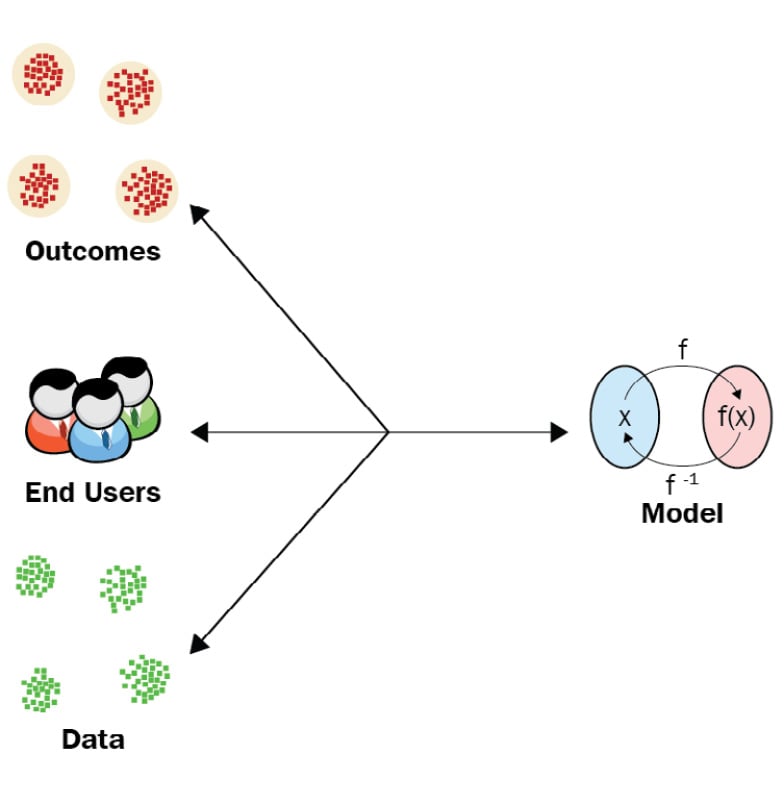
Figure 1.7 – Four dimensions of explainability
Now, let's learn about these dimensions in detail:
- Data: The dimension of explainability revolves around the underlying data that is being modeled. Understanding the data, identifying its limitations and relevant components, and forming certain hypotheses are crucial to setting up the correct expectations. A robust data curation process, analyzing data purity, and the impact of adversarial effects on the data are other key exercises done for obtaining explainable outcomes.
- Model: Model-based interpretability techniques often help us to understand how the input data is mapped to the output predictions and be aware of some limitations and assumptions of the ML algorithms used. For example, the Naïve Bayes algorithm used for ML classification assumes that the presence of a certain feature is completely independent and unrelated to the presence of any other features. So, knowing about these inductive biases of ML algorithms helps us to understand and anticipate any prediction error or limitations of the ML models.
- Outcomes: The outcome of explainability is about understanding why a certain prediction or decision is made by an ML model. Although data and model interpretability is quite crucial, most ML experts and end-users focus on making the final model predictions interpretable.
- End users: The final dimension of explainability is all about creating the right level of abstraction and including the right amount of details for the final consumers of the ML models so that the outcomes are reliable and trustworthy for any non-technical end-user and empower them to understand the decision-making process of black-box algorithms.
Explainability to AI/ML algorithms is provided with respect to one or more dimensions of explainability. Next, let's discuss about addressing the key questions of explainability.
Addressing key questions of explainability
Now that we understand the different dimensions of explainability, let's discuss what is needed to make ML models explainable. In order to make ML algorithms explainable, the following are the key questions that we should try to address:
- What do we understand from the data?
The very first step is all about the data. Before even proceeding with the AI and ML modeling, we should spend enough time analyzing and exploring the data. The goal is always to look for gaps, inconsistencies, potential biases, or hypotheses that might impact or create challenges while modeling the data and generating the predictions. This helps us to know what is expected and how certain aspects of the data can contribute toward solving the business problem.
- How is the model created?
We need to understand how transparent the algorithm is and what kind of relationship the algorithm can capture when modeling the data during the modeling process. This is the step where we try to understand the inductive bias of the algorithms and then try to relate this to the initial hypothesis or observations obtained while exploring the data. For example, linear models will not model the data efficiently if the data has some quadratic or cycle patterns observed using visualization-based data exploration methods. The prediction error is expected to be higher. So, if it is unclear how the algorithm builds a model of the training data, these algorithms are less transparent and, hence, less interpretable.
- What do we know about the global interpretability of the trained model?
Understanding the global model interpretability is always challenging. It is about getting a holistic view of the underlying features used, knowing the important features, how sensitive the model is toward changes in the key feature values, and what kind of complex interactions are happening inside the model. This is especially hard to achieve in practice for complex deep learning models that have millions of parameters to learn and several hundreds of layers.
- What is the influence of different parts of the model on the final prediction?
Different parts of an ML model might impact the final prediction in a different way. Especially for deep neural network models, each layer tries to learn different types of features. When model predictions are incorrect, understanding how different parts of a model can affect or control the final outcome is very important. So, explainability techniques can unravel insights from different parts of a model and help debug and observe the algorithm's robustness for different data points.
- Why did the model make a specific prediction for a single record and a batch of records?
The most important aspect of explainability is understanding why the model is making a specific prediction and not something else. So, certain local and global explanation techniques are applied, which either consider the impact of individual features or even the collective impact of multiple features on the outcome. Usually, these explainability techniques are applied for single instances of the data and a batch of data instances to understand whether the observations are consistent.
- Does the outcome match the expectation of the end user?
The final step is always providing user-centric explanations. This means explainability is all about comparing the outcome with end users' predictions based on common sense and human intuition. If the model forecast matches the user's prediction, providing a reasonable explanation includes justifying the dominant factors for the specific outcome. But suppose the model forecasting is not matching the user's prediction. In that case, a good explanation tries to justify what changes could have happened in the input observations to get a different outcome.
For example, let's say, considering usual weekday traffic congestion, the time taken to reach from office to home for me is 30 minutes. But if it is raining, I would expect the vehicles on the road to move slowly and traffic congestion to be higher, and hence might expect it to take longer to reach home. Now, if an AI application predicts the time to get home as still 30 minutes, I might not trust this prediction as this is counter-intuitive.
Now, let's say that the algorithm was accurate in its forecast. However, the justification provided to me was about the movement of the vehicles on my route, and the AI app just mentioned that the vehicles on my route are moving at the same speed as on other days. Does this explanation really help me to understand the model predictions? No, it doesn't. But suppose the application mentions that there are fewer vehicles on the route than found on typical days. In that case, I would easily understand that the number of vehicles is fewer due to the rain and hence the time to destination is still the same as usual on weekdays.
My own recommendation is that, after training and validating an ML model, always try to seek answers to these questions as an initial step in interpreting the working of black-box models.
Understanding different types of explanation methods
In the previous section, we discussed some key questions to address when designing and using robust explainability methods. In this section, we will discuss various types of explanation methods, considering the four dimensions of explainability used in ML:
- Local explainability and global explainability: ML model explainability can be done for single local instances of the data to understand how a certain range of values or specific categorical value can be related to the final prediction. This is called local explainability. Global model explainability is used to explain the behavior of the entire model or certain important features as a whole that contribute toward a specific set of model outcomes.
- Intrinsic explainability and extrinsic explainability: Some ML models, such as linear models, simple decision trees, and heuristic algorithms, are intrinsically explainable as we clearly know the logic or the mathematical mapping of the input and output that the algorithm applies, whereas extrinsic or post hoc explainability is about first training an ML model on given data and then using certain model explainability techniques separately to understand and interpret the model's outcome.
- Model-specific explainability and model-agnostic explainability: When we use certain explainability methods that are applicable for any specific algorithm, then these are model-specific approaches. For example, visualization of the tree structure in decision tree models is only specific to the decision tree algorithm and hence comes under the model-specific explainability method. Model-agnostic methods are used to provide explanations to any ML model irrespective of the algorithm being used. Mostly, these are post hoc analysis methods, used after the trained ML model is obtained, and usually, these methods are not aware of the internal model structure and weights. In this book, we will mostly focus on model-agnostic explainability methods, which are not dependent on any particular algorithm.
- Model-centric explainability and data-centric explainability: Conventionally, the majority of explanation methods are model-centric, as these methods try to interpret how the input features and target values are being modeled by the algorithm and how the specific outcomes are obtained. But with the latest advancement in the space of data-centric AI, ML experts and researchers are also investigating explanation methods around the data used for training the models, which are known as data-centric explainability. Data-centric methods are used to understand whether the data is consistent, well curated, and well suited for solving the underlying problem. Data profiling, detection of data and concept drifts, and adversarial robustness are certain specific data-centric explainability approaches that we will be discussing in more detail in Chapter 3, Data-Centric Approaches.
We will discuss all these types of explainability methods in later chapters of the book.
Understanding the accuracy interpretability trade-off
For an ideal scenario, we would want our ML models to be highly accurate and highly interpretable so that any non-technical business stakeholder or end user can understand the rationale behind the model predictions. But in practice, achieving highly accurate and interpretable models is extremely difficult, and there is always a trade-off between accuracy and interpretability.
For example, to perform radiographic image classification, intrinsically interpretable ML algorithms, such as decision trees, might not be able to give efficient and generalized results, whereas more complex deep convolutional neural networks, such as DenseNet, might be more efficient and robust for modeling radiographic image data. But DenseNet is not intrinsically interpretable, and explaining the algorithm's working to any non-technical end user can be pretty complicated and challenging. So, highly accurate models, such as deep neural networks, are non-linear and more complex and can capture complex relationships and patterns from the data, but achieving interpretability is difficult for these models. Highly interpretable models, such as linear regression and decision trees, are primarily linear and less complex, but these are limited to learning only linear or less-complex patterns from the data.
Now, the question is, is it better to go with highly accurate models or highly interpretable models? I would say that the correct answer is, it depends! It depends on the problem being solved and on the consumers of the model. For high-stake domains, where the consequences of poor predictions are severe, I would recommend going for more interpretable models even if accuracy is being sacrificed. Any rule-based heuristic model that is highly interpretable can be very effective in such situations. But if the problem is well studied, and getting the least prediction error is the main goal (such as in any academic use case or any ML competitions) such that the consequences of poor prediction will not create any significant damage, then going for highly accurate models can be preferred. In most industrial problems, it is essential to keep the right balance of model accuracy and interpretability to promote AI adoption.
Figure 1.10 illustrates the accuracy-interpretability trade-off of popular ML algorithms:
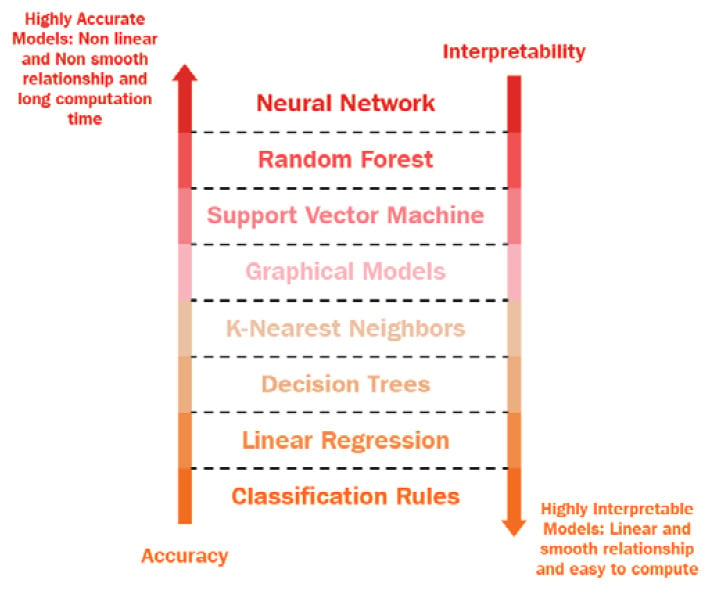
Figure 1.8 – Accuracy-interpretability trade-off diagram
Now that we have a fair idea of the accuracy-interpretability trade-off, let's try to understand how to evaluate the quality of explainability methods.