Evaluating the quality of explainability methods
Explainability is subjective and may vary from person to person. The key question is How do we determine whether one approach is better than the other? So, in this section, let's discuss certain criteria to consider for evaluating explainability techniques for ML systems.
Criteria for good explainable ML systems
As explainability for ML systems is a very subjective topic, first let's try to understand some key criteria for good human-friendly explanations. In his book Interpretable Machine Learning, Christoph Molnar, the author, has also tried to emphasize the importance of good human-friendly explanations after thorough research, which I will try to mention in a condensed form considering modern, industrial, explainable ML systems:
- Coherence with a priori knowledge: Consistency with prior beliefs of end users is an important criterion of explainable ML systems. If any explanation contradicts a priori knowledge of human beings, then humans tend to have less trust in such explanations. However, it is challenging to introduce prior knowledge of humans into ML models. But human-friendly explainable ML systems should try to provide explanations surrounding certain features that have direct and less complex relationships with the outcome, such that the relationship is coherent with the prior beliefs of the end users.
For example, for predicting the presence of diabetes, the measure of blood glucose level has a direct relationship, which is consistent with prior human beliefs. If the blood glucose level is higher than usual, this might indicate diabetes for the patient, although diabetes can also be due to certain genetic factors or other reasons. Similarly, high blood glucose levels can also be momentary and a high blood glucose level doesn't always mean that the patient has diabetes. But as the explanation is consistent with a priori knowledge, end users will have more trust in such explanations.
- Fidelity: Another key factor for providing a holistic explanation is the truthfulness of the explanation, which is also termed the fidelity of ML models. Explanations with high fidelity can approximate the holistic prediction of the black-box models, whereas low-fidelity explanations can interpret a local data instance or a specific subset of the data. For example, for doing sales forecasting, providing explanations based on just the trend of historical data doesn't give a complete picture, as other factors, such as production capacity, market competition, and customer demand, might influence the outcome of the model. Fidelity plays a key role, especially for doing a detailed root cause analysis, but too many details may not be useful for common users, unless requested.
- Abstraction: Good human-friendly explanations are always expected in a concise and abstract format. Too many complicated details can also impact the experience of end users. For example, for weather forecasting, if the model predicts a high probability of rain, the concise and abstract explanation can be that it is cloudy now and raining within 5 kilometers of the current location, so there is a high probability of rain.
But if the model includes details related to precipitation level, humidity, and wind speed, which might also be important for the prediction of rainfall, these additional details are complex and difficult to comprehend, and hence not human-friendly. So, good, human-friendly explanations include the appropriate amount of abstraction to simplify the understanding for the end users. End users mostly prefer concise explanations, but detailed explanations might be needed when doing root cause analysis for model predictions.
- Contrastive explanations: Good, human-friendly explanations are not about understanding the inner workings of the models but mostly about comparing the what-if scenarios. Suppose the outcome is continuous numerical data as in the case of regression problems. In that case, a good explanation for predictions includes comparing with another instance's prediction that is significantly higher or lower. Similarly, a good explanation for classification problems is about comparing the current prediction with other possible outcomes. But contrastive explanations are application-dependent as it requires a point of comparison, although understanding the what-if scenarios helps us to understand the importance of certain key features and how these features are related to the target variable.
For example, for a use case of employee churn prediction, if the model predicts that the employee is likely to leave the organization, then contrastive explanations try to justify the model's decision by comparing it with an instance's prediction where the employee is expected to stay in the organization and compare the values of the key features used to model the data. So, the explanation method might convey that since the salary of the employee who is likely to leave the organization is much lower than that of employees who are likely to stay within the organization, the model predicted that the employee is expected to leave the organization.
- Focusing on the abnormality: This may sound counter-intuitive, but human beings try to seek explanations for events that are not expected and not obvious. Suppose there is an abnormal observation in the data, such as a rare categorical value or an anomalous continuous value that can influence the outcome. In that case, it should be included in the explanation. Even if other normal and consistent features have the same influence on the model outcome, still including the abnormality holds higher importance in terms of human-friendly explanation.
For example, say we are predicting the price of cars based on their configuration, let's say the mode of operation is electric, which is a rare observation compared to gasoline. Both of these categories might have the same influence on the final model prediction. Still, the model explanation should include the rare observation, as end users are more interested in abnormal observations.
- Social aspect: The social aspect of model explainability determines the abstraction level and the content of explanations. The social aspect depends on the level of understanding of the specific target audience and might be difficult to generalize and introduce during the model explainability method. For example, suppose a stock-predicting ML model designed to prescribe actions to users suggests shorting a particular stock. In that case, end users outside the finance domain may find it difficult to understand. But instead, if the model suggests selling a stock at the current price without possessing it and buying it back after 1 month when the price is expected to fall, any non-technical user might comprehend the model suggestions easily. So, good explanations consider the social aspect, and often, user-centric design principles of Human-Computer Interaction (HCI) are utilized to design good, explainable ML systems that consider the social aspect.
Now that we have a fair idea of the key criteria for good explanations, in the next section, we will discuss some auxiliary criteria that are equally important while building explainable ML systems.
Auxiliary criteria of XAI for ML systems
Good explanations are not limited to the key criteria discussed previously, but there are a few auxiliary criteria of XAI as discussed by Doshi-Velez and Kim in their work Towards A Rigorous Science of Interpretable Machine Learning:
- Unbiasedness: Model explainability techniques should also look for the presence of any form of bias in the data or the model. So, one of the key goals of XAI is to make ML models unbiased and fair. For example, for predicting credit card fraud, the explainability approach should investigate the importance of demographic information related to the gender of the customer for the model's decision-making process. If the importance of gender information is high, that means that the model is biased toward a particular gender.
- Privacy: Explainability methods should comply with data privacy measures, and hence any sensitive information should not be used for the model explanations. Mainly for providing personalized explanations, ensuring compliance with data privacy can be very important.
- Causality: Model explainability approaches should try to look for any causal relationships so that the end users are aware that due to any perturbation, there can be changes in model predictions for production systems.
- Robustness: Methods such as sensitivity analysis help to understand how robust and consistent a model prediction is with respect to its feature values. If small changes in input features lead to a significant shift in model predictions, it shows that the model is not robust or stable.
- Trust: One of the key goals of XAI is to increase AI adoption by increasing the trust of the end users. So, all explainability methods should make black-box ML models more transparent and interpretable so that the end users can trust and rely on them. If explanation methods don't meet the criteria of good explanations, as discussed in the Criteria for good explainable ML systems section, it might not help to increase the trust of its consumers.
- Usable: XAI methods should try to make AI models more usable. Hence, it should provide information to the users to accomplish the task. For example, counterfactual explanations might suggest a loan applicant pays their credit card bill on time for the next 2 months and clear off their previous debts before applying for a new loan so that their loan application is not rejected.
Next, we will need to understand various levels of evaluating explainable ML systems.
Taxonomy of evaluation levels for explainable ML systems
Now that we have discussed the key criteria for designing and evaluating good explainable ML systems, let's discuss the taxonomy of evaluation methods for judging the quality of explanations. In their work Towards A Rigorous Science of Interpretable Machine Learning, Doshi-Velez and Kim mentioned three major types of evaluation approaches that we will try to understand in this section. Since explainable ML systems are to be designed with user-centric design principles of HCI, human beings evaluating real tasks play a central role in assessing the quality of explanation.
But human evaluation mechanisms can have their own challenges, such as different types of human bias and being more time- and other resource-consuming, and can have other compounding factors that can lead to inconsistent evaluation. Hence, human evaluation experiments should be well designed and should be used only when needed and otherwise not. Now, let's look at the three major types of evaluation approaches:
- Application-grounded evaluation: This evaluation method involves including the explainability techniques in a real product or application, thus allowing the conduction of human subject experiments, in which real end users are involved to perform certain experiments. Although the experiment setup cost is high and time-consuming, building an almost finished product and then allowing domain experts to test has its benefits. It will enable the researcher to evaluate the quality of the explanation with respect to the end task of the system, thus providing ways to quickly identify errors or limitations of the explainability methods. This evaluation principle is consistent with the evaluation methods used in HCI, and explainability is infused within the entire system responsible for solving the user's keep problem and helping the user meet its end goal.
For example, to evaluate the quality of explanation methods of an AI software for automated skin cancer detection from images, dermatologists can be approached to directly test the objective for which the AI software is built. If the explanation methods are successful, then such a solution can be scaled up easily. In terms of the industrial perspective, since getting a perfect finished product can be time-consuming, the better approach is to build a robust prototype or a Minimum Viable Product (MVP) so that the domain expert testing the system gets a better idea of how the finished product will be.
- Human-grounded evaluation: This evaluation method involves conducting human subject experiments with non-expert novice users on more straightforward tasks rather than domain experts. Getting domain experts can be time-consuming and expensive, so human-grounded evaluation experiments are easier and less costly to set up. The tasks are also simplified sometimes and usually for certain use cases where generalization is important, these methods are very helpful. A/B testing, counterfactual simulations, and forward simulations are certain popular evaluation methods used for human-grounded evaluation.
In XAI, A/B testing provides different types of explanations to the user, where the user is asked to select the best one with the higher quality of explanation. Then, based on the final aggregated votes, and using other metrics such as click-through rate, screen hovering time, and time to task completion, the best method is decided.
For counterfactual simulation methods, human subjects are presented with the input and output of the model with the model explanations for a certain number of data samples and are asked to provide certain changes to the input features in order to change the model's final outcome to a specific range of values or a specific category. In the forward simulation method, human subjects are provided with the model inputs and their corresponding explanation methods and then asked to simulate the model prediction without looking at the ground-truth values. Then, the error metric used to find the difference between human-predicted outcomes with the ground-truth labels can be used as a quantitative way to evaluate the quality of explanation.
- Functionality-grounded evaluation: This evaluation method doesn't involve any human subject experiments, and proxy tasks are used to evaluate the quality of explanation. These experiments are more feasible and less expensive to set up than the other two, and especially for use cases where human subject experiments are restricted and unethical, this is an alternative approach. This approach works well when the type of algorithm was already tested in human-level evaluation.
For example, linear regression models are easily interpretable and end users can efficiently understand the working of the model. So, using a linear regression model for use cases such as sales forecasting can help us to understand the overall trend of the historical data and how the forecasted values are related to the trend.
Figure 1.9 summarizes the taxonomy of the evaluation level for explainable ML systems:
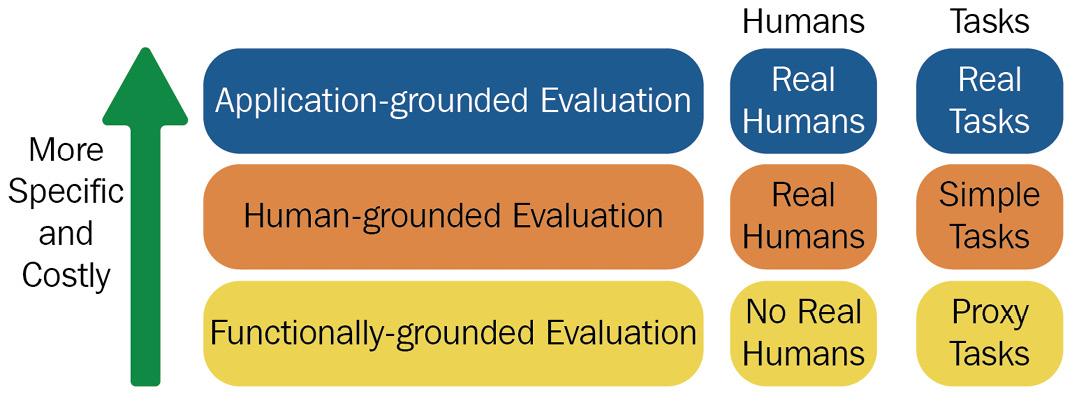
Figure 1.9 – Taxonomy of evaluation level for the explainable ML system
Apart from the methods discussed here, for determining the quality of explanations, other metrics such as description length of explanation, the complexity of the features used in the explanation, and cognitive processing time required to understand the provided explanation, are often used. We will discuss them in more detail in Chapter 11, End User-Centered Artificial Intelligence.
In this chapter, so far you have come across many new concepts for ML explainability techniques. The mind-map diagram in Figure 1.10 gives a nice summary of the various terms and concepts discussed in this chapter:
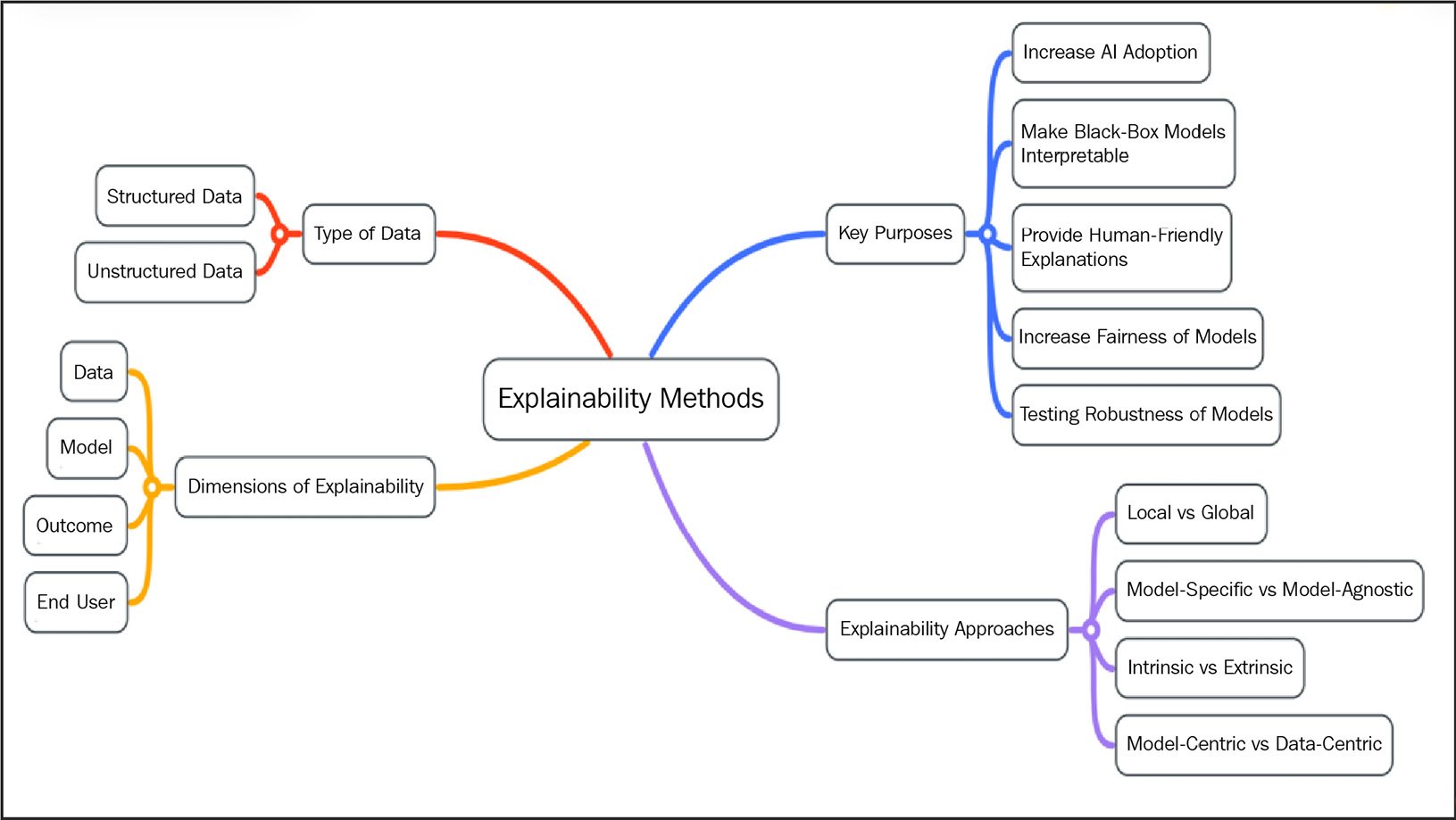
Figure 1.10 – Mind-map diagram of machine learning explainability techniques
I strongly recommend all of you make yourselves familiar with the jargon used in this mind-map diagram as we will be using it throughout the book! Let's summarize what we have discussed in the summary section.