Solving scheduling errors
At this point, you may have already experienced some issues with scheduling pipelines not being triggered as expected. If not, don’t worry; it will happen sometime and is totally normal. With several pipelines running in parallel, in different windows, or attached to different timezones, it is expected to be entangled with one or another.
To avoid this entanglement, in this exercise, we will create a diagram to assist in the debugging process, identify the possible causes of a scheduler not working correctly in Airflow, and see how to solve it.
Getting ready
This recipe does not require any technical preparation. Nevertheless, taking notes and writing down the steps we will follow here can be helpful. Writing when learning something new can help to fix the knowledge in our minds, making it easier to remember later.
Back to our exercise; scheduler errors in Airflow typically give the DAG status None
, as shown here:
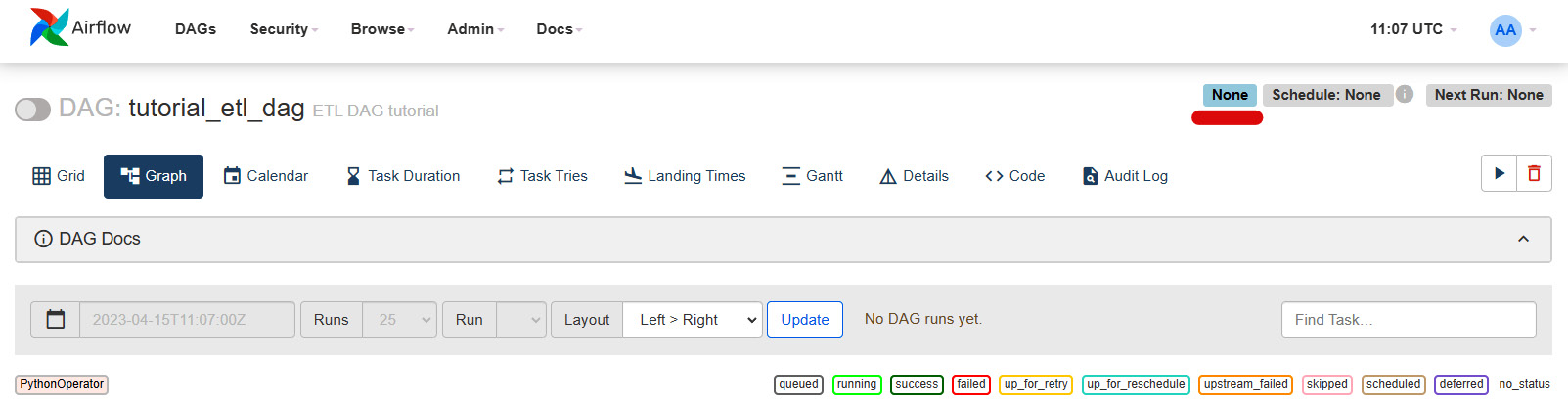