Before we go in depth into how decision tree algorithms work, let's examine their outcome in more detail. The goal of decision trees is to extract from the training data the succession of decisions about the attributes that explain the best class, that is, group membership.
In the following example of the conditional inference tree, we try to predict survival (there are two classes: Yes
and No
) in the Titanic
dataset we used in the previous chapter. Now to simplify things, there is an attribute called Class
in the dataset. When discussing the outcome we want to predict (the survival of the passenger), we will use a lowercase c
(class), and when discussing the Class
attribute (with 1st, 2nd, 3rd, and Crew), we will use a capital C
. The code to generate the following plot is provided at the end of the chapter, when we describe conditional inference trees:
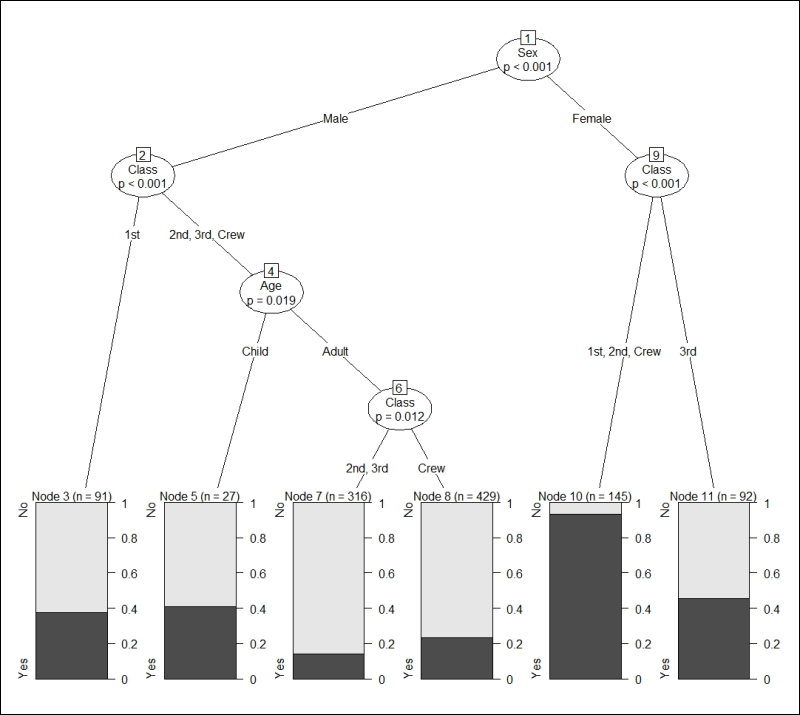
Example of decision tree (conditional inference tree)
Decision trees have a root (here: Sex), which is the best...