Samuel Arthur, known to be the father of machine learning, defines it as a field of study that gives computers the ability to learn without being explicitly programmed. To simplify it, machine learning is a scientific discipline that explores the construction and study of algorithms that can learn from data. Such algorithms operate by building a model from example inputs and use that model to make predictions or decisions rather than following strictly static program instructions.
To illustrate, consider that you have a dataset that contains the information about age, education, gender, and annual income of a sufficiently large number of people. Suppose you are interested in predicting someone's income. So, you will build a model by choosing a machine learning algorithm and train the model with the dataset. After you train your model, it can then predict the income of a new person if you provide it with age, education, and gender data. To explain it further, you have not programmed something explicitly, such as if a male's age is greater than 50 and whether he has a master's degree, then he would earn say $100,000 per annum. However, what you did was just choose a generic algorithm and gave it the data, so that it discovers all the relationships between the different variables or features (here, age, gender, and education) with the target variable income. So, the algorithm learned from the data and hence got trained. Now, with the trained algorithm, you can predict someone's income if you know their other variables.
The preceding example is a typical kind of machine learning problem where there exists a target variable or class; here that is income. So, the algorithm learns from the training data or examples and then after being trained, the algorithm predicts for a new case or data point. Such learning is known as the Supervised Machine Learning. It works as shown in the following figure:
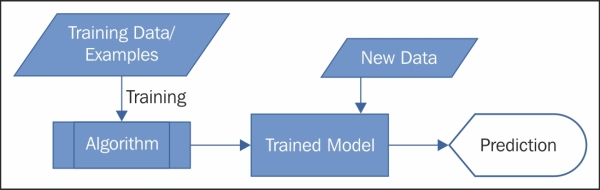
There is another kind of machine learning where there is no target variable or the concept of training data or examples, so here, the prediction is also of a different kind. Consider the same dataset again that contains data of age, gender, education, and income of a sufficiently large number of people. You have to run a targeted marketing campaign, so you have to divide or group the people into three clusters. In this case as well, you can use a different kind of machine learning generic algorithm on the dataset that would automatically group the people into three groups or clusters. This kind of machine learning is known as unsupervised machine learning.
There is also another kind of machine learning that makes recommendations; remember how Amazon recommends books or Netflix recommends movies—which might surprise you as to how magically they know about a user's choice or taste.
Though machine learning is not limited to these three kinds, for the scope of this book, we would limit it to these three.
Again, the scope of this book and, of course, Azure Machine Learning limits the application of machine learning to just the area of predictive analytics only. You should be aware that machine learning is not limited to this. Machine learning finds it roots in artificial intelligence and powers a variety of applications, some of which you use in everyday life, for example, web search engines, such as Bing or Google are powered by Machine Learning or applications, so also personal digital assistants like Microsoft's Cortana and Apple's Siri. These days, driverless cars are also in the news, which use machine learning. So, such applications are countless.
The following are some of the common kinds of problems solved through machine learning.
Classification is the kind of machine learning problem where inputs are divided into two or more classes and the learner produces a model that assigns unknown inputs to one (or multi-label classification) or more of these classes or labels. This is typically handled in a supervised way. Spam detection is an example of classification, where the inputs or examples are e-mail (or other) messages and the classes are "spam" and "not spam" and the model to predict a new e-mail as spam or not are based on example data.
Regression problems involve predicting a numerical or continuous value for the target variable for the new data given in the dataset with one or more features or dependent variables and associated target values. A simple example can be where you have historical data of the price paid for different properties in your locality for say the last 5 years. Here, the price paid is the target variable and the different attributes of a property, such as the total built-up area; the type of property, such as a flat or semi-detached house; and so on, are different features or variables. A regression problem would be to predict the property price of a new property available in the market for sale.
Clustering is an unsupervised learning problem and works on a dataset with no label or class variable. This kind of algorithm takes all of the data and groups them into different clusters say 1, 2, and 3, which were not known previously. The clustering problem is fundamentally different from the classification problem. The classification problem is a supervised learning problem where your class or target variable is known to train a dataset, whereas in clustering, there is no concept of label and training data. It works on all the data, and groups them into different clusters.
So, to put it simply, if you have a dataset and a class/label or target variable as a categorical variable, and you have to predict the target variable for a new dataset based on the given dataset (example), then this is a classification problem. If you are just given a dataset with no label or target variable and you just have to group them into n clusters, then it's a clustering case.
The following are some of the very popular machine learning algorithms:
Linear regression is probably the most popular and classic statistical technique used for regression problems to make prediction for a continuous value from one or more variables or features. This algorithm uses a linear function and it optimizes the coefficients that fit best to the training data. If you have only one variable, then you may think of this model as a straight line that best fits the data. For more features, this algorithm optimizes best hyperplane that fits the training data.
Logistic regression is a statistical technique used for classification problems. It models the relationship between a dependent variable or a class label and independent variables (features) and then makes a prediction of a categorical dependent variable or a class label. You may think of this algorithm as a linear regression for a classification problem.
A decision tree is a set of questions or decisions and their possible consequences arranged in a hierarchical fission. While the plain decision tree is not very powerful, an assembly of trees with the averaged out results can be very effective. These are ensemble models and differ by how the decision is sampled or chosen. Random forest or decision forest and boosted decision tree are two very popular and powerful algorithms. Decision tree-based algorithms can be used for both classification and regression problems.
Neural networks algorithms are inspired by how a human brain works. It builds a network of computation units, neurons, or nodes. In a typical network, there are three layers of nodes: first, the input layer, the middle layer or hidden layer, and in the end, the output layers. Neural networks algorithms can be used for both classification and regression problems.
A special kind of neural networks algorithms where there are more than three layers along with the input and output layers and more than one hidden layers are known as Deep learning algorithms. These are getting increasingly popular these days because of remarkable results.
Though Azure Machine Learning is capable of deep learning (convolutional neural network—a flavor of the deep learning model as of writing of this book), the book does not include it.