Thus far, we have assumed that the internal training structure of PPO mirrors what we learned when we first looked at neural networks and DQN. However, this isn't actually the case. Instead of using a single network to derive Q values or some form of policy, the PPO algorithm uses a technique called actor–critic. This method is essentially a combination of calculating values and policy. In actor–critic, or A3C, we train two networks. One network acts as a Q-value estimate or critic, and the other determines the policy or actions of the actor or agent.
We compare these values in the following equation to determine the advantage:

However, the network is no longer calculating Q-values, so we substitute that for an estimation of rewards:

Now our environment looks like the following screenshot:
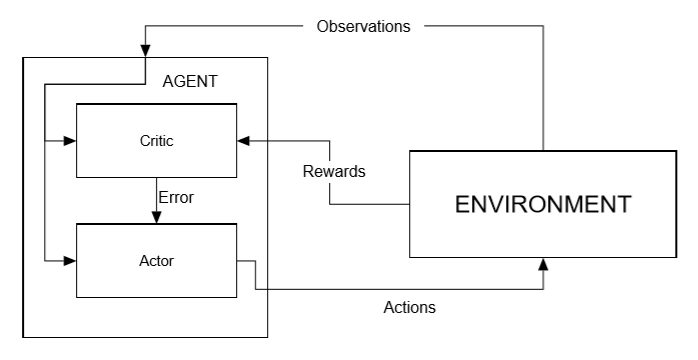
Diagram of actor–...