Conditional random fields (CRFs)
BiLSTM models look at a sequence of input words and predict the label for the current word. In making this determination, only the information of previous inputs is considered. Previous predictions play no role in making this decision. However, there is information encoded in the sequence of labels that is being discounted. To illustrate this point, consider a subset of NER tags: O, B-Per, I-Per, B-Geo, and I-Geo. This represents two domains of person and geographical entities and an Other category for everything else. Based on the structure of IOB tags, we know that any I- tag must be preceded by a B-I from the same domain. This also implies that an I- tag cannot be preceded by an O tag. The following diagram shows the possible state transitions between these tags:
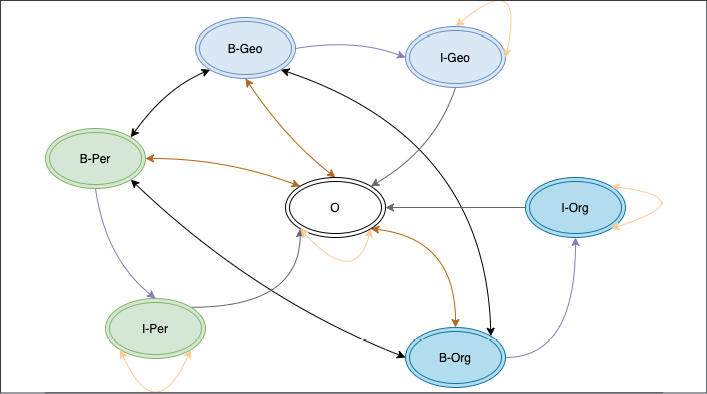
Figure 3.2: Possible NER tag transitions
Figure 3.2 color codes similar types of transitions with the same color. An O tag can transition only to a B tag...