Using Azure Machine Learning datasets
During this chapter, we have covered what an Azure Machine Learning datastore is and how to connect to a variety of supported data sources. We created connections to Azure Machine Learning datastores using Azure Machine Learning Studio, the Python SDK, and the Azure CLI. We have just covered Azure Machine Learning datasets, a valuable asset for your ML projects. We went through how to generate Azure Machine Learning datasets using Azure Machine Learning Studio and the Python SDK. Once an Azure Machine Learning dataset is created, it can be used throughout your Azure Machine Learning experiments, which are called jobs.
Figure 2.21 shows a code snippet for materializing the mltable
artifact into a pandas dataframe. Please note that you need the mltable
library installed in your environment (using the pip install
mltable
command).
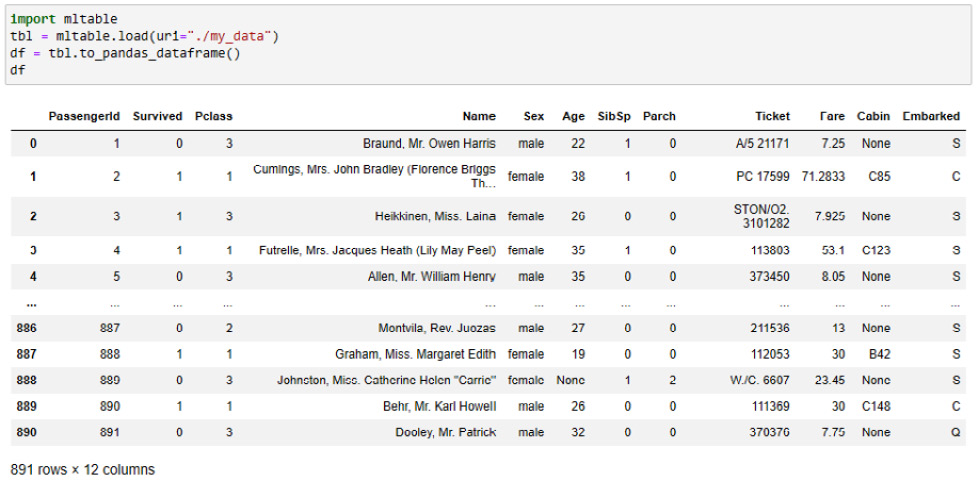
Figure 2.21 – Materializing the mltable artifact into a pandas dataframe
Now let...